Is AI the existential threat we think it is, set to replace all human UX researchers? Or can designers and researchers harness and incorporate its mind-boggling capabilities into their work?
In 2023, global AI market revenue was $196.6 billion. Over the next five to ten years, that number is expected to grow at 37% annually. Or 31%. Or 19%, depending on your source.(1)(2)(3)
Our point is, AI’s influence is already BIG. And it’s only going to get BIGGER.
It’s forced a rethink of research and design roles, and the organizational design and dynamics that surround them.
In this article, we’ll explore fundamental concepts of AI and how it’s permeating the UX Research and Design fields.
Here’s a tl;dr summary of what we’ll cover:
- Understanding AI in UX Research
- Enhanced Data Collection and Analysis
- Personalization and Tailored Experiences
- Capabilities that AI brings to the research table
- Using Advanced Research in Research Analytics and Design
- Drawbacks of using generative AI in UX research
- Key factors to consider while developing any new technology
- Advice from experts at Google and Microsoft about using AI in research
- Chatbots, Virtual Assistants and Conversational Interfaces
- Extracting Actionable Insights
- Future Trends and Innovations
- AI’s Transformative Impact on UX Research
Understanding AI in UX Research
Ever notice how colleagues at a new job use abbreviations completely alien to you?
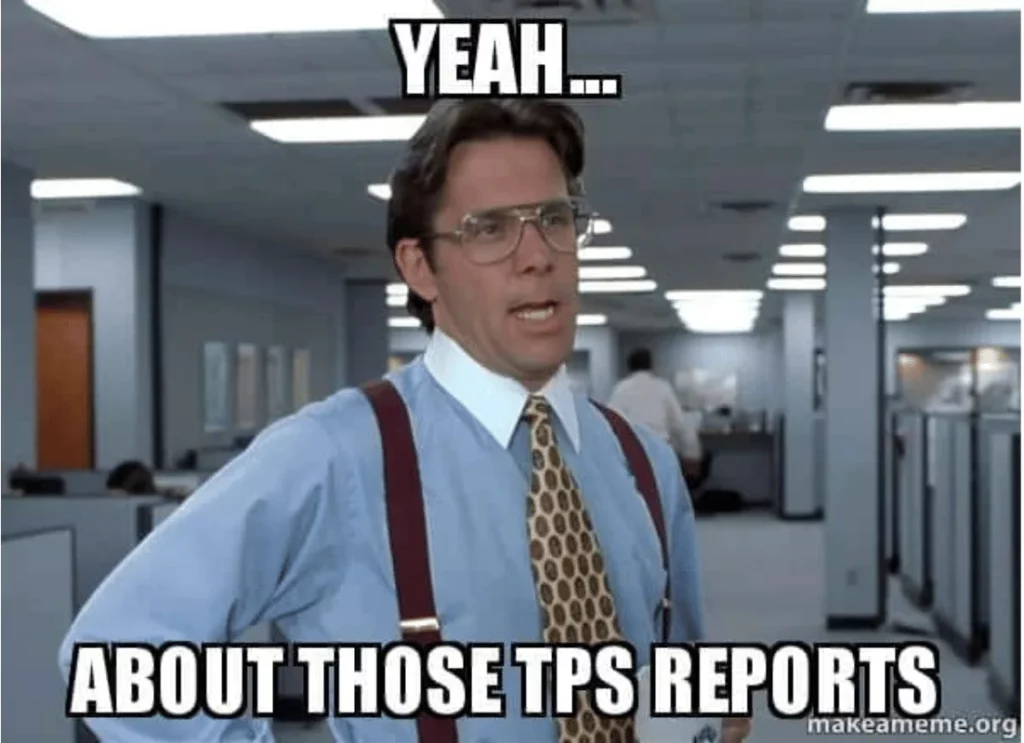
Worry not. When it comes to fundamental AI, we’ve got you covered. Use the table below to stay well-versed with basic AI concepts and terminology:
[Forewarning: “Abr” stands for abbreviation. We did it again. Sigh.]
Marvin’s Guide to AI Terminology
Phrase | Abr. | Description |
Artificial Intelligence | AI | Umbrella term for the simulation of human intelligence by a machine. Intelligence = perceiving, synthesizing and inferring information. |
Machine Learning | ML | Subset of AI, ML uses algorithms that enable computers to learn patterns or make predictions based on a dataset. |
Natural Language Processing | NLP | Type of AI that conducts interactions between humans and computers. Machines understand, interpret and generate human-esque responses. Initially trained with real-life data, they use pattern recognition to come up with responses. |
Large Language Models | LLM | AI algorithm that uses language as their input and output. Trained on extremely large datasets to understand, summarize, generate and predict new content. |
Generative AI | GenAI | Type of AI that can create images, video, audio, text and 3-D models. |
Application Programming Interface | API | Software that facilitates communication between different applications. |
Neural Network Model | NNM | AI model that teaches computers to process data like a human brain, so it can make decisions on its own. Unlike ML (which makes decisions based on what it learned from data). |
Generative Pre-Trained Transformers | GPT | Series of neural network models that learn context and meaning by tracking relationships in sequential data. Think about how auto-complete knows what to write next in messaging apps. |
Learn about the capabilities and limitations of AI in UX research.
Now, let’s examine how these various technologies fit into a researcher’s or designer’s workflow.
Enhanced Data Collection and Analysis
Two approaches to data collection here — passive and active. Active studies are conducted to answer a specific research question. Passive data collection involves collecting data on an ongoing basis.
To collect data from active research projects, Marvin’s AI uses NLP and LLM to transcribe your user interviews. Get a verbatim transcript within minutes. No more frantic note-taking.
Learn more about the differences between passive and active research.
Let’s talk about passive data collection. The prevalence of APIs means that apps (Google Analytics and the like) supply a continuous data stream to companies. Once in a research repository, ML helps researchers unearth trends and patterns from the dataset. AI tools for data analysis ensure that critical insights don’t go overlooked.
Researchers can use AI to conduct rapid analysis, processing volumes of data from complex datasets in real-time. The benefits are threefold:
- Aggregating and interpreting user data in real-time brings adaptability. In an ever-evolving digital landscape, it helps researchers unearth “in-the-moment” insights. These insights provide companies with feedback for on-the-fly decision-making, eliminating guesswork.
- AI’s ability to handle large volumes of data enables researchers to take on more extensive projects without increasing the time and resources spent.
- Another upside is scalability. AI allows researchers to meet increased data processing needs (previously impossible if conducted by humans). AI allows companies to ramp up their research efforts rapidly and efficiently.
AI can collect and analyze data round the clock for you — 24 hours a day, 7 days a week…you get the idea.
Learn more tips and tricks to incorporate the use of AI in your research.
Personalization & Tailored Experiences
Ever scrolled through a friend’s YouTube feed? You likely noticed it’s entirely different from yours. A prime example of a customized experience.
UX will always be centered around designing an experience that delights each and every user. Designers and researchers’ primary aim is to create tailored experiences that improve customer:
- Adoption
- Engagement
- Satisfaction
- Retention
Using data about how users interact with an application, AI can decipher individual user patterns and preferences. To craft well-rounded user personas, AI uses NLP to understand people’s attitudes and emotions from social media posts, forums, and customer reviews.
AI is capable of dynamically adapting interfaces and suggestions based on these user personas.
This opens up the door for more inclusivity and accessibility. Using AI-driven tools, researchers and designers can create applications and processes that cater to a more diverse user base, considering their abilities, backgrounds and general product use. UX researchers can then study the effectiveness of personalized features and iterate on design choices to improve user satisfaction.
AI will eventually tailor experiences to each individual user. This results in highly customized user experiences based on an individual’s preferences.
Imagine a day when ALL your apps look and feel completely different from someone else’s. With AI, that level of personalization is right around the corner.
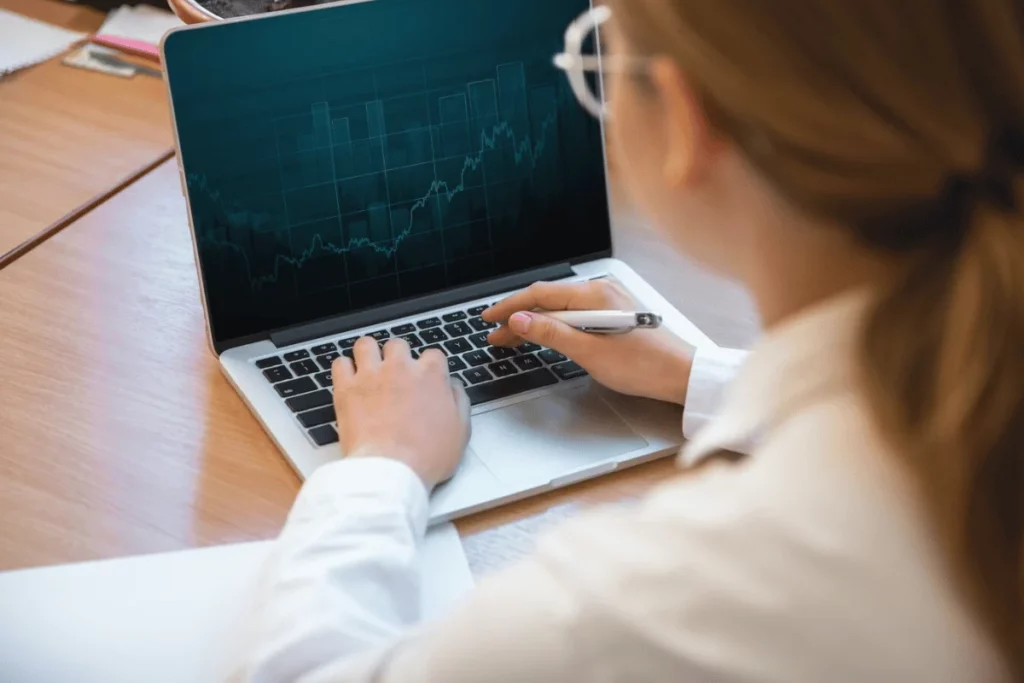
Using Advanced AI in Research Analytics and Design
Ever wondered how Amazon knows exactly when you’re out of dishwasher tablets or detergent?
It’s down to predictive analytics.
Amazon’s AI examines your consumption and purchase history, and it uses ML to estimate when you’ll run out. That’s when it automatically sends you the eerie notification.
Predictive analytics uses statistical algorithms and ML techniques to enable a deeper understanding of user behavior. Designers can identify patterns in data and troubleshoot user issues or pain points to improve UX design. It enables UX professionals to make better-informed design decisions.
AI analytics can alert professionals to anomalies and issues. However, it still requires a human being to interpret results and understand their implications.
AI has forged its way into visual design as well. AI features expedite design tasks. It can help create a plethora of prototypes or wireframes that weren’t humanly possible before.
Companies such as Adobe have introduced AI features to help designers build visually appealing and effective user interfaces. To name a few:
- Visual asset analysis — comparing attention across various creative elements
- Text-to-image generation
- Color & font pairing
- Cropping or resizing
- Removal of items/objects
Implementing advanced AI in research analytics and design results in well-designed user interfaces. AI can also help gather and analyze usability metrics to understand what’s working and what isn’t. It makes the feedback cycle a lot shorter.
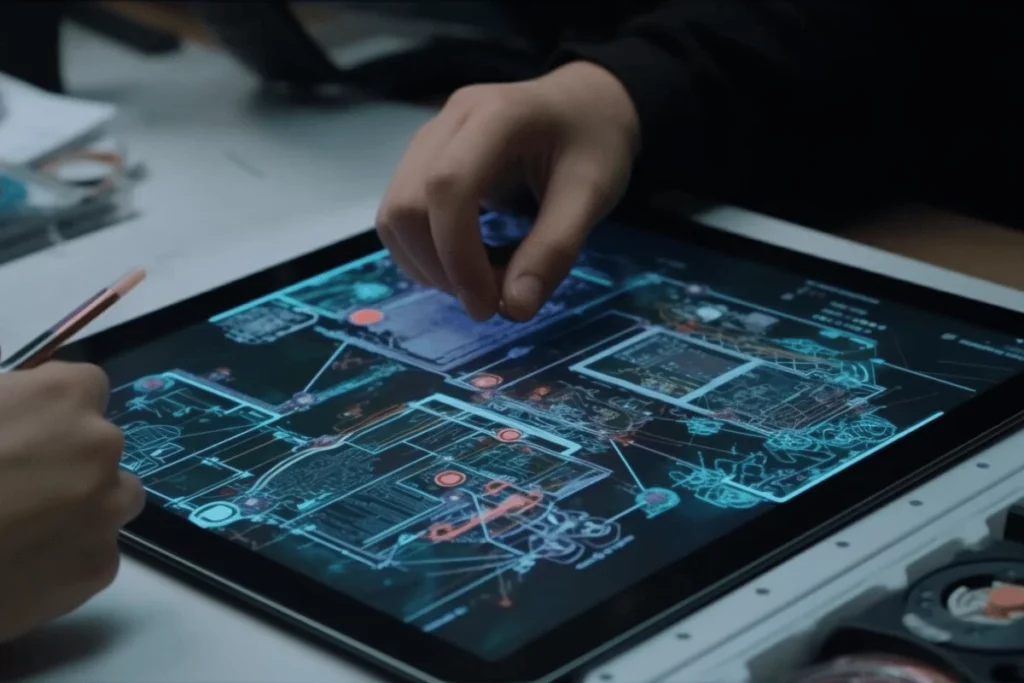
Chatbots, Virtual Assistants and Conversational Interfaces
ChatGPT’s release was revolutionary. It changed how college students worked (or avoided work), how we access information, and brought efficiency to the workplace.
Our friends at User Interviews conducted a study with over 1,000 UX professionals and found that 77% of respondents already use some form of AI in their work.
NLP is the underlying technology behind applications like ChatGPT. It’s essential for chatbots, voice assistants (such as Siri or Alexa), and sentiment analysis. UX researchers can leverage NLP to conduct surveys and analyze user feedback. This allows them to iterate and improve the conversational aspect of a user interface.
If you haven’t jumped on the AI chatbot bandwagon already, FAQPrime assembled a beginner’s list of prompts to get you started. Tinker with these commands to see what suits your workflow best. Remember to follow these basic guidelines to follow when interacting with a chatbot or virtual assistant:
- Provide ample context
- Ask for multiple options
- Iterate on the output
- Build a prompt library
Search Your Own Research the Way You Search the Web
Marvin’s Ask AI product is the ChatGPT of Research. Trying to garner insights from old studies? A perennial pain in the behind, no more! Ask any question to begin searching your research repository and get the answers you need in seconds.
Aside from transcribing calls, Marvin’s end-to-end research assistant conducts foundational qualitative analysis for you. It creates automatic notes during and after interviews and is capable of articulating the gist of mundane and long conversations with AI generated summaries. AI synthesis allows researchers to annotate on the fly.
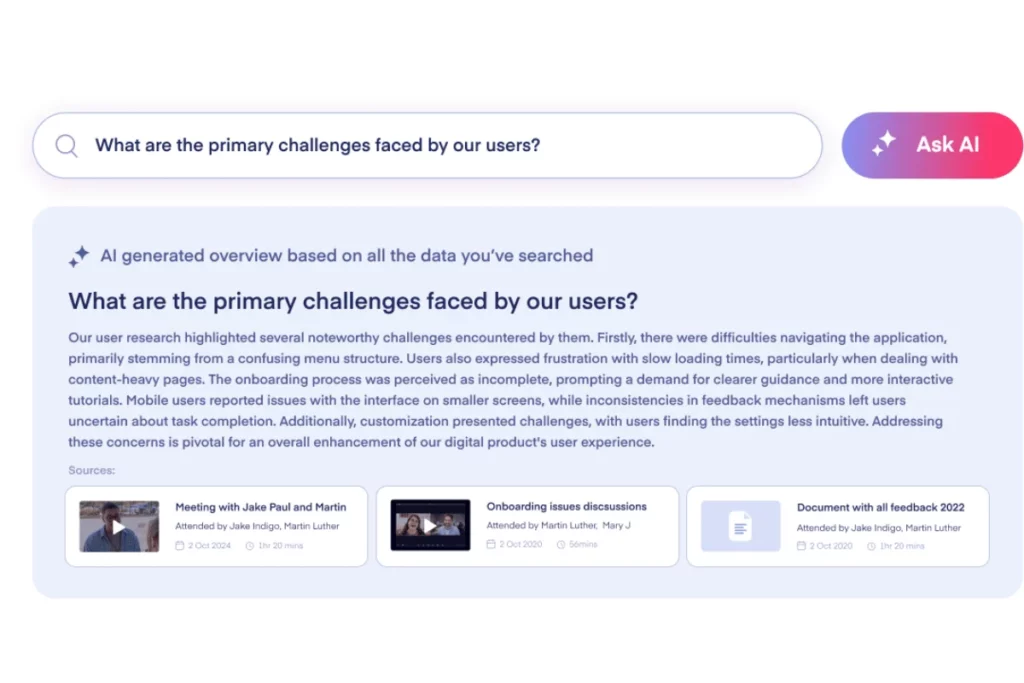
*Marvin UX Research Products visual of AI Research Assistant
AI frees up a researcher’s time to focus fully on the interview and delve deeper into user pain points. Remember, using AI as a sidekick is like working with an intern. While fully capable of understanding instructions and completing tasks, you still have to check their work.
Learn about Marvin’s most popular products launched in 2023.
Extracting Actionable Insights
With AI’s help, designers can conduct comprehensive design reviews. Observing how users interact with a system (or predicting how they will interact) provides insight into the user journey. This allows researchers and designers to scrutinize UIs to identify usability issues and other areas that need improvement.
AI’s ability to handle vast and complex datasets accelerates the shift from raw data to actionable insights. AI extracts patterns and insights much faster than a human would. Using AI to conduct preliminary analysis and synthesis creates an immediacy in results. This helps answer questions like:
What changes can we make to the product based on this information?
It all leads to the development of designing smarter, modular design interfaces that are user-friendly.
Data-driven decision-making improves the accuracy and quality of research. It provides insights using real-time data to identify areas of interest. Researchers and designers can then make informed choices based on the underlying data.
AI adds efficiency to the design process. However, its findings or results need to be interpreted by someone(human). You still (and always will) need a human being on hand to establish the direction of research and make sense of it all.
The Good: Benefits of AI in UX Research
Following its $10 billion investment into OpenAI*, Microsoft announced an upcoming AI co-pilot soon to be deployed in Microsoft’s Office suite. Co-pilot boasts the ability to quickly analyze data, generate reports and create presentations (among many). Working professionals (including yours truly) were left in limbo, worrying for their long term job prospects.
Sounds too close to home? Let us alleviate your fears.
Microsoft called it “co-pilot” implying someone else is flying the plane: You.
AI may be ground-breaking technology, but at the end of the day, it’s a technology that exists to serve humans. As directors of technology, it becomes increasingly important for us to understand how to effectively use AI during analysis, and for what. Learn how AI can become your ultimate UX research sidekick (or your copilot or the Robin to your Batman or… you get the idea).
Optimize UX Workflow
Qualitative research provides a wholesome understanding of phenomenon through rich insights. Arriving at those insights involves a tedious, cumbersome data annotation process called coding or tagging.
Traditionally, UX researchers toiled away, spending hours transcribing and tagging interview data. Not anymore. Research tools like Marvin use analytical AI to create transcripts of virtually any interview recording you throw at them. By automating these mechanical tasks, it frees up a researcher’s time to focus wholly on the participant and conduct deeper analysis. Marvin users spend 60% less time analyzing UX research data. Set your research up for success — explore our guide on how to use tags to reach insights faster.
One thing that’s indisputable is that AI is bound to make workers more efficient. With AI in their toolkit, UX researchers and product designers will spend less time wrestling with tricky and convoluted data, and more time on analysis.
Enable Large-Scale Analysis with AI in UX Research
Improved efficiency unlocks the door to conduct data analysis en masse. Deconstructing an interview transcript is a complex process. Researchers can suffer from the dreaded data overload — there’s simply too much to unpack from unstructured data from individual interviews and group sessions, verbal and non-verbal cues and overlapping themes.
AI facilitates the analysis of vast amounts of data — sit back and let it pore through mountains of historical data. It has the ability to predict possible customer behavior and evaluate how they interact with designs. The amount of predictive analysis AI is capable of was not physically or mentally possible before the introduction of AI (not by a human, anyway!).
Start your analysis on the right foot. Marvin uses generative AI to create synopses of interviews, condensing key points from hours of interview time into a paragraph or two. Far preferable to get a quick gist than reading a twenty page long transcript. Marvin’s AI generates auto-notes for your recordings. A solid structure and foundation lays the path for deeper analysis. By delegating the heavy lifting to AI, researchers can do more with all this newfound time on their hands.
[DISCLAIMER: We encourage you not to think of the output as the final product, but a starting point for your analysis.]
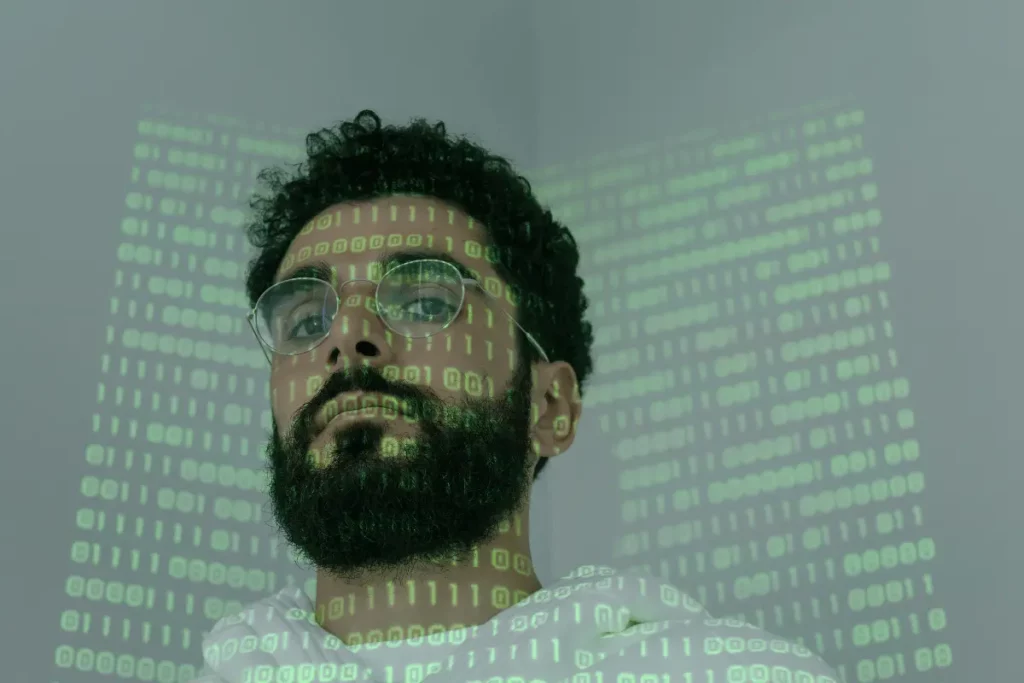
Deliver Consistency & Reliability
What machines do better than humans, is follow instructions to the tee. Researchers can define an interpretive grid (a coding scheme) and set AI models to work, so that they perform some initial heavy lifting. Any failure cases based on the algorithm can then be further explicated.
Bias is inherent in every study — unfortunately that’s attributable to the researcher. AI can be trained and corrected to interpret and eradicate bias. This makes studies more equitable across the board.
Privacy is a fundamental right. Protecting user privacy is of utmost importance while conducting user research. We obsess over protecting participants’ Personal and Identifiable Information (PII) such as their names, contact details, gender and occupation. We teamed up with Assembly AI to pioneer a first-of-its-kind PII redaction model that automatically strips away participant PII from audio and video files. Powered with AI; more peace of mind for everyone.
Uncover New Patterns with AI in UX Research
Analytical AI has the capacity to unearth unexpected and interesting insights. Given the right prompt, it can detect patterns and themes in textual data, and generate insights that even researchers may have missed.
AI levels the playing field enabling multilingual analysis and promoting cultural diversity in research. With text mining & natural language processing (NLP), research tools like Marvin can translate and transcribe numerous languages. This breaks down barriers to communication, overcoming language limitations, form, styles and varying academic conventions.
With AI, a refreshing and novel idea may be just round the corner.
Facilitate Collaboration
AI originally found itself catering to fields of computer science and engineering, but now permeates through various disciplines. It now transcends healthcare, business and finance, psychology and neuroscience.
At Marvin, we wanted a platform to centralize all user insights, so they all live in one place. No more duplicative efforts, harness the power of a centralized research repository. We also want users across disciplines to continue with existing tools that they already use. Share playlists, clips and insights with your peers. Whether they’re researchers or not – everyone benefits from first-hand feedback from end users.
LiveNotes, our collaborative note taking tool, enables you to create time-stamped insights, so you can quickly bookmark and annotate important parts of an interview while conducting it. Your findings are always accessible and editable upon later viewing — synthesize them live or post with colleagues. Integrate your video conferencing platform of choice (Zoom, Meet, Teams) and document your observations with your peers.
Our core values ring true: Elevate the user voice across your organization. Create a customer-centric culture.
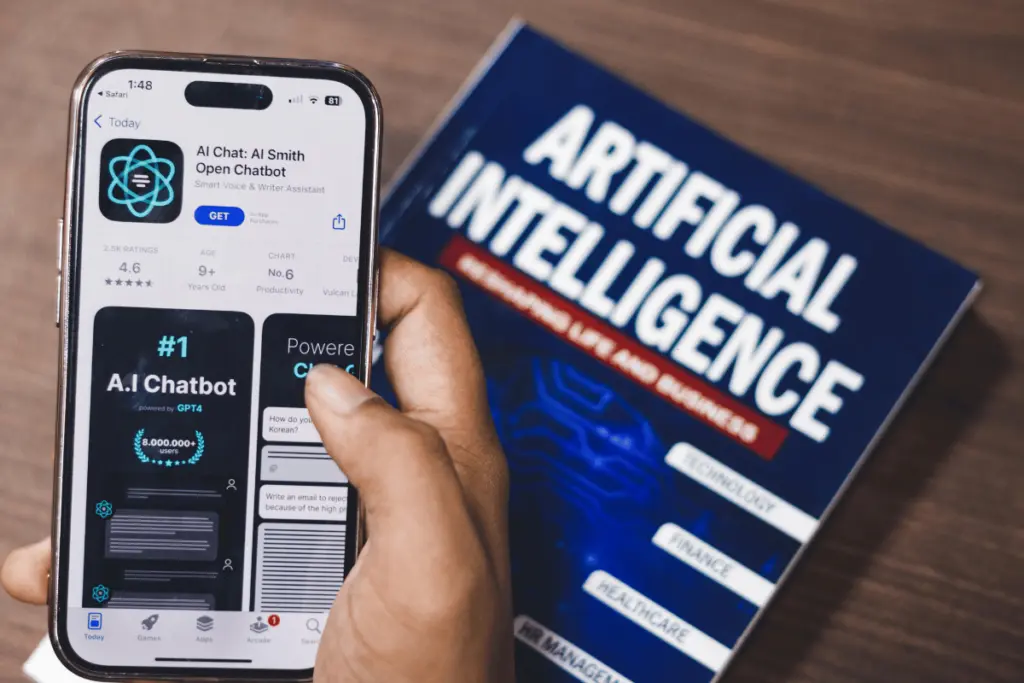
The Bad: Risks of AI in UX Research
On the road to implementing new tech, there’s bound to be rough and bumpy bits along the way. AI will only get better with each iteration — Chat GPT-4 is far more advanced and capable than its predecessors.
Call them usability enhancements or bug fixes, the fact is we learn more about the nuances of a particular application with time as we use it. It’s vital to understand the limitations of AI in UX research work — drawing the line under what it can help you with versus what it can’t.
Baked-in Bias
Bias is a double-edged sword. We’re all biased, whether we care to admit it or not. Above, we looked at how AI can reduce human bias by automating certain procedures. Consider this — any AI model is coded by a developer. Developers may unknowingly and inadvertently bake in bias into their model, resulting in skewed analysis and reinforcing their own existing social prejudices, stereotypes or inequalities.
Plenty of recent examples illustrate how big tech companies failed to detect inherent bias in their systems. Companies such as Amazon, Microsoft and Google have been in the news for the wrong reasons — their AI algorithms unknowingly exhibited racial and gender bias.
Google Research Scientist Rida Qadri recently conducted a study on the (poor) representation of South Asian cultures in text-to-image AI models. Rida suggests that since these models are based on the internet as a collective archive of information, they are largely representative of the western world. As a result, South Asian cultures are often poorly represented, if at all.
There are serious ethical considerations to take into account when developing new AI technologies. Researchers must delve deeper into AI output and seek to identify and correct any biases. Award-winning researcher Mary Gray questions whether we are failing certain groups in society. She touts qualitative research as key to building more ethically responsible AI.
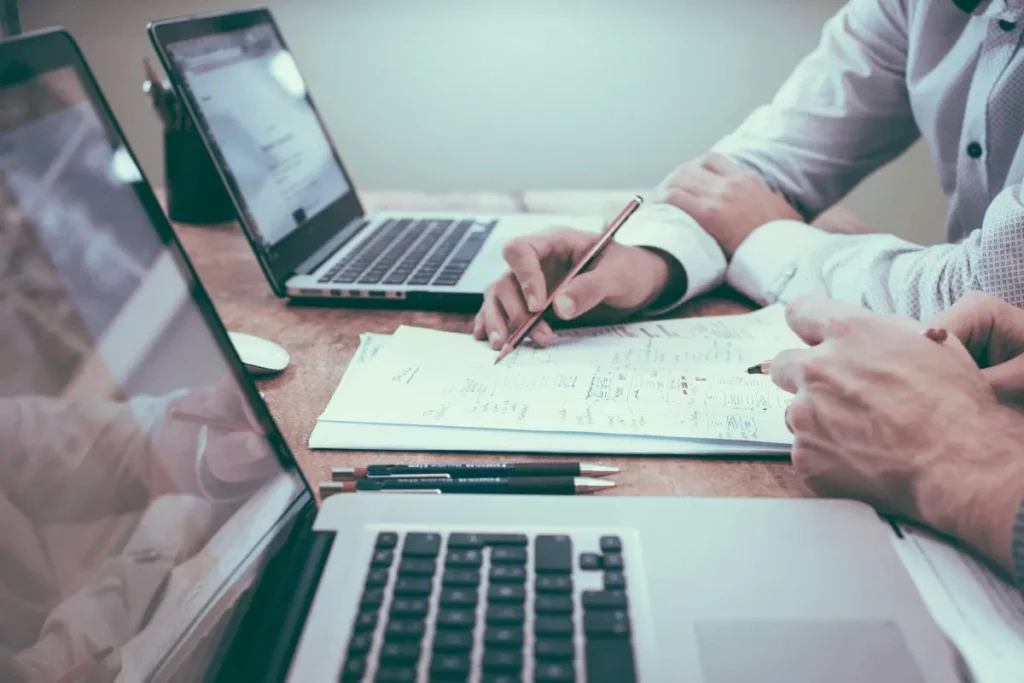
Less Context
How does AI perform in providing context in your qualitative insights?
Check out these two studies:
Here’s a quick tl;dr recap:
Actual human researchers faced off with a machine to unearth qualitative insights from an open-ended question. While the machine took considerably less time to conduct its analysis, the human analysis was more in-depth and comprehensive. The machine’s categorizations were simplistic and unhelpful. In a sentiment analysis, machines missed the crux of participant responses by isolating each word individually, failing to group them into coherent themes at all.
As humans, the researchers had an understanding of the question and the reasoning behind it. While classifying responses, they can be relied on to provide the right context, group phrases together and tag data intuitively.
We understand other humans — what their responses mean and what they are alluding to. That’s what separates man from machine.
Lost Human Touch
“AI is limited by its inability to possess human-level understanding of the social world” – Hubert. L Dreyfus, 1992.
There’s no getting around it — the responsibility of creating a study and all conclusions drawn from it will always rest with human researchers. No matter how comprehensive results or insights are, AI cannot be accorded with any ownership or authorship of research. AI has no common sense, an inability to learn from experience and a lack of understanding of social and cultural nuances.
Qualitative research relies a lot on forming inferences from an interaction. When you interview a participant, you choose how to navigate the interview, forming new questions and interpretations along the way.
AI lacks that human touch — the intuition and experience that a lifetime of interactions has given us. We spoke earlier about giving interviewees your full attention – AI wouldn’t pick up on subtleties such as a change in facial expression or a shift in the tone of voice.
Humans can gauge sentiment. Fidelity’s VP of Design Ben Little spoke to us about how craftsmanship is making a comeback. A techno-optimist, Ben says that the mass adoption of AI will only increase the novelty of human-crafted design. There are some things you can’t replace; AI just doesn’t have lived experience, imagination and empathy — three inherent human traits.
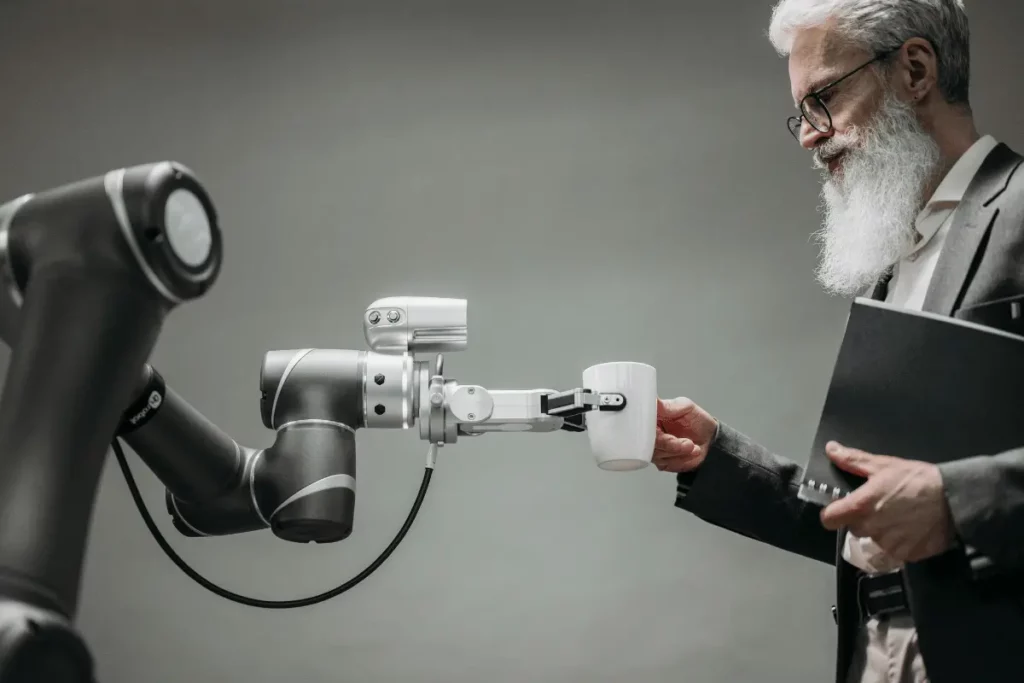
Noisy Data
Ever heard of GIGO? A computer science wordplay on the concept of the first-in first-out (FIFO) inventory accounting method (yawn), it stands for “Garbage in, garbage out.”
Quite simply, what you put into something, you get out of it. We’re not in the business of doling out life lessons, but the same logic applies to any AI model. The quality of output is dependent on the quality of input. Put garbage in, and don’t be surprised when garbage comes out.
Ask any data analyst, product designer or researcher — the bulk of their time is spent cleaning and sanitizing data. Output from AI models are dependent on pre-processed, accurate data. AI struggles to deal with inconsistent or ambiguous data.
Mary talked passionately about ensuring models have a complete and comprehensive dataset to begin with. Rida explained that this isn’t so easy. It’s hard to shake off the indelible Western influence over technology. Since AI uses the web as the foundational library of all information, it’s important to remember that it’s not all-encompassing.
Continually ask yourself — are we training AI models on the right dataset?
Overreliance on Technology
Should you ask any youngster today a general knowledge or simple math question, watch them consult their smartphones before their brain. Spend a day at work without your phone and observe how disconnected and naked you feel. Surprise, surprise, we’re all a bit too reliant on technology.
This cuts the same way for UX researchers. If AI is doing all the work for you, your neurons aren’t likely firing on all cylinders. Going through the motions, researchers can miss insights, lose interest in the automated tasks which diminishes their critical thinking and analytical skills.
Mechanization culls creativity. Design is an artistic field, a realm of imagination and innovation. Leaning too heavily on tech can make us lazy, complacent and boring. Does churning out something that’s been done to death work?
Likely not.
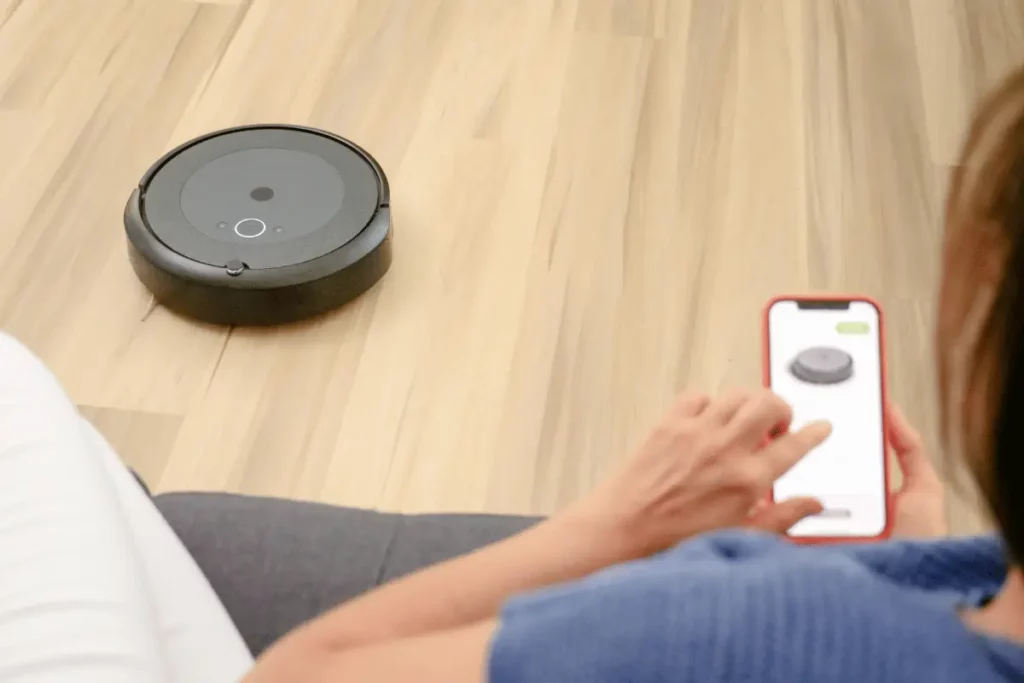
Future Trends and Innovations
AI will augment researchers’ work, not replace researchers themselves.
We’re more bullish on this assertion today than ever before.
Marvin CEO Prayag Narula believes AI is the perfect research assistant. AI-generated summaries help with a superficial understanding of the big picture. They also save researchers precious time ordinarily spent rewatching long videos and taking notes.
Our users tells us Marvin’s AI transcription is game-changing. It frees up their time and mindspace to focus on deepening their understanding of users.

AI in UX Research Technology to Watch For
However, transcripts miss important context — they don’t capture emotion. People don’t verbalize all their actions and describe everything that they’re thinking.
To tackle these shortcomings, some AI tech is still in the works:
- Biometric technology is (theoretically) capable of capturing human emotion from video or visual artifacts. Its current output is confusing, messy, and misleading — it requires more training data. Emotion recognition has the potential to provide valuable insights into users.
- Synthetic users are largely unpopular among the UX community. Designers argue that they build for humans, not synthetic or artificial users. However, there are instances when it can be useful. During early days of a research practice, it can help smaller teams increase the scale of their work.
With AI technology developing rapidly, it might be a short time before these features are added to its growing arsenal.
To understand more about the UX industry’s continued evolution, Lattice’s VP of Design stopped by to share his predictions for 2024. Jared reminded researchers and designers to focus on:
- Efficiency — or how to do more with less. Companies will harness a combination of researchers, designers, and AI capabilities to maximize research output.
- AI literacy is now a prerequisite. It moves from being a “nice-to-have” to a “must-have” skill for researchers and designers. Companies will look to hire UX professionals who are proficient or at least competent in AI.
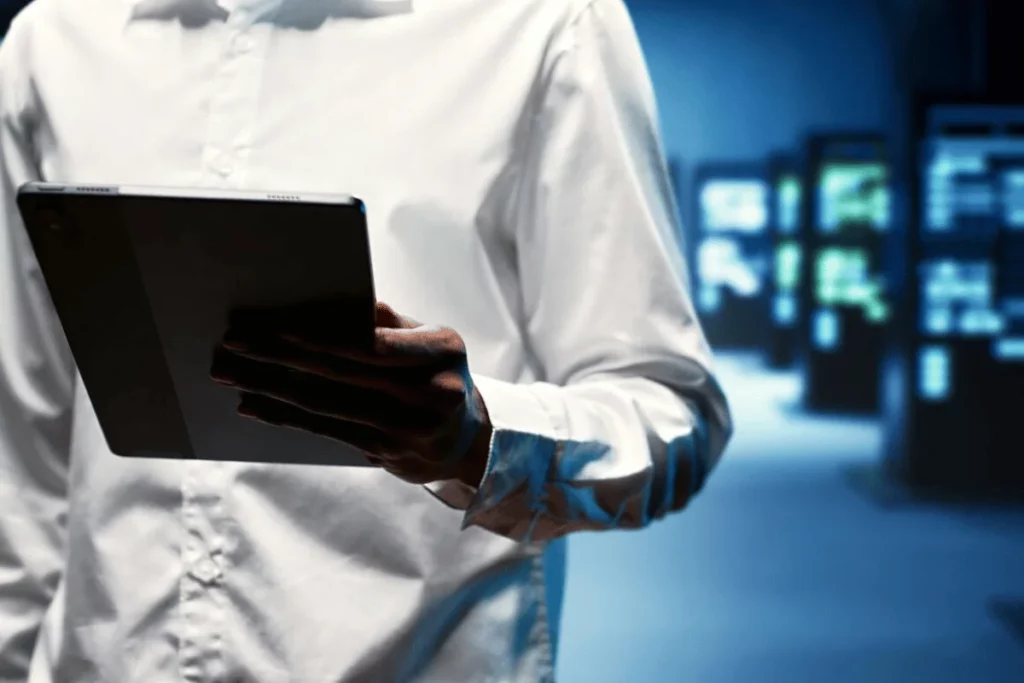
The Final Verdict of AI in UX Research
[Admittedly, we dropped the ball with the title of this section. We wanted to replicate the Clint Eastwood western classic, but we couldn’t paint the future as ‘ugly’. Read on to find out why.]
What does the future hold for UX researchers and AI? We’re quite bullish on AI in research. Our two cents (literally) is two factors to constantly keep in mind as you build and introduce new products into the world:
Consider the Community Impact
Technology has a transformational impact on society. Computers used to occupy the size of a room, but now sit in our pockets. With a few swipes, you can have groceries delivered home, learn a new language or hail a taxi.
Rida Qadri examined how technology is continually shaped by social contexts. She spent time in Jakarta examining mobility platforms and how they adapted to the existing mobility landscape. Uber revolutionized travel and mobility in the West, but their approach doesn’t translate across the globe. As designers, we don’t get to tell users how to use our technology. Noone can predict user behavior.
We may have the best intentions in releasing groundbreaking AI technologies. However, we would be doing communities and wider humanity a disservice if we don’t spend a substantial amount of time trying to understand the impact AI will have on these communities.
Try understanding the impact these technologies have not on the immediate, but wider communities, even ones you might not be examining today.
Maintain User-Centric Design
Throughout the product design process, the most important questions designers must ask themselves are:
- Why are we building this?
- What problem(s) does it solve for our customers?
- What are any potential challenges we may encounter?
This is the crux of establishing user empathy. Never lose sight of these core questions, constantly circle back to them.
And remember, a customer-centric culture is not something that AI can build for you.
Learn more tips and tricks to incorporate the use of AI in your research.
In a Nutshell: AI in UX Research Will Make Us Better Researchers
There’s no reason why UX professionals and AI can’t enjoy a peaceful co-existence. AI helps facilitate rapid and thorough analysis, giving designers and researchers the gift of time. It comes with its fair share of limitations, which must be understood fully while using it. As with any nascent technology, plenty of kinks to be ironed out.
Central to leveraging AI will always be the human behind it. Technology is here to serve humans, not the other way around. The rise of AI is not necessarily about replacing researchers and designers, but empowering them to become more productive. Think of AI less as a threat and more of an apparatus in the UX toolkit, one that supercharges your productivity.
Future Trends and Innovations
AI will augment researchers’ work, not replace researchers themselves.
We’re more bullish on this assertion today than ever before.
Marvin CEO Prayag Narula believes AI is the perfect research assistant. AI-generated summaries help with a superficial understanding of the big picture. They also save researchers precious time ordinarily spent rewatching long videos and taking notes.
Our users tell us Marvin’s AI transcription is game-changing. It frees up their time and mind space to focus on deepening their understanding of users.
Learn more about G2’s top-rated UX research repository
Find out how to integrate Marvin’s AI features into your UX research workflow.
Book a free demo today.
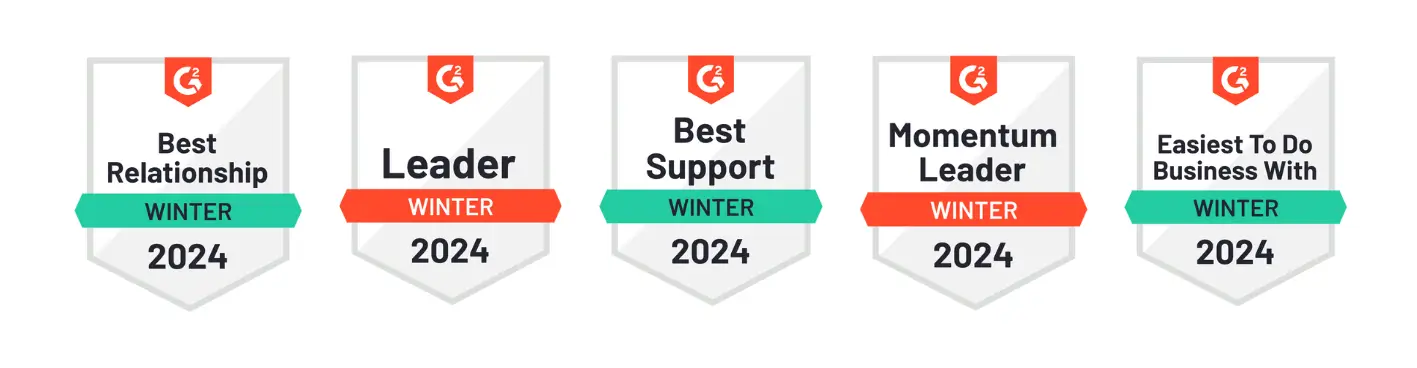
*Quick note that Sam Altman is one of Marvin’s lead investors. We’re big fans of his work and grateful for his support of our user research platform!