The importance of user testing isn’t lost on us.
Once deemed a luxury, it’s now a necessity. Companies today conduct testing at every stage of design and development. To create beautiful products and services, designers must get continuous feedback. Only then can they iterate on designs, and deliver what users want. AI technologies will shape the way future designers and researchers work. It will also revolutionize the user testing process.
Let’s explore how designers, developers and researchers can use AI to automate parts of the testing process. This frees up their time for more creative work and strategic innovation.
A Breakdown of Common User Testing Techniques
Designers strive to create experiences that delight users.
User testing allows designers to gather valuable feedback from their target audience. It provides insight into how people interact with a product or service. Companies track how customers navigate through an interface. This uncovers pain points or usability issues. They extract meaningful insights about user preferences and behavior.
This helpful graphic below showcases the different types of user testing:
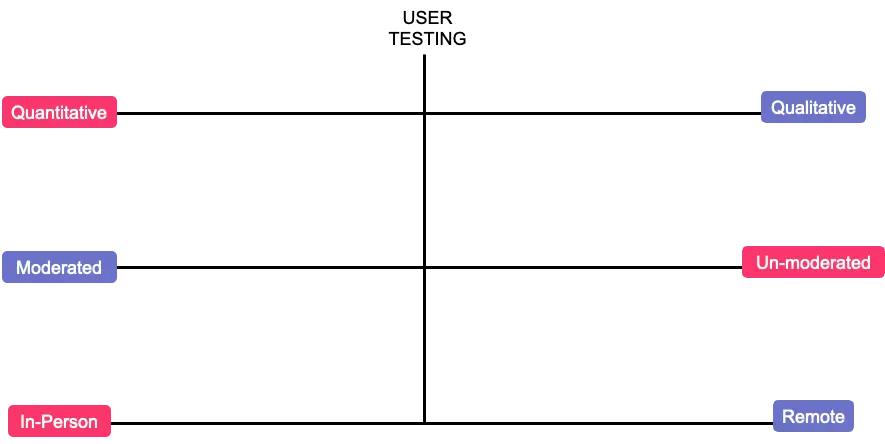
- To get a holistic understanding of the user experience, research must include a mix of quantitative and qualitative data.
- Trained moderators control the testing process. They guide participants through tasks and navigation. When users encounter unexpected roadblocks or problems, moderators gather feedback. Unmoderated testing takes place without supervision. The test just needs to be created and distributed. Participants can run through it on their own time.
- Remote testing happens from anywhere. All that’s required is an internet connection. Users can share screens and record their activity, while providing feedback. In-person testing involves a researcher and participants in the same location. These tests are more time consuming and expensive.
Companies amass vast amounts of data from testing. All in the name of improving the UX, customer satisfaction and retention.
Automated Testing with AI in UX
Traditional user testing is a manual and human-intensive process. Researchers carry out everything. From creating a test script, through to execution and post-game analysis. Human error (and in all likelihood, bias) is unavoidable.
Automated testing tools use AI to write test scripts. They distribute and execute tests, and can generate a report summarizing the findings. Every task performed is code and script-based, so there’s no potential for human error. This makes it more reliable than manual testing. It also saves time. A LOT of time.
Read on for more benefits and efficiency gains that AI can bring to the user testing process…
Benefits of Automated Testing with AI
- Big Data Handling. AI is capable of analyzing big data from various sources. Perfect for companies looking to collect large swathes of data on a regular basis. AI streamlines development processes, by consistently applying the same methodology to its tasks. AI can work round-the-clock and provides real-time insights for faster decision making. It goes about its business with greater efficiency and less errors than a human. With automation, companies can scale up their data collection and processing. This allows them to make data-driven design decisions.
- Resource Optimization. We’re talking about the big 3 — people, money and time. Unmoderated tests can run virtually unattended. Intelligent automation engines collect and analyze data 24×7. AI removes manual analysis, which is tedious and time consuming. Without worrying about data processing, researchers can concentrate on more strategic endeavors. They can refine product features, address critical issues and continue to innovate. In the long run, companies who replace manual research with AI automation will save on the big 3.
- Greater Coverage. We don’t mean to sound like AT&T or Verizon. AI allows testing of various user behavior scenarios (statements, branches and paths). Classification algorithms help developers select user paths from an infinite number of possibilities. AI continuously learns from data, selecting different user journeys from ones previously executed. Automated testing can handle a multitude of test cases across environments. This ensures that the product performs as intended under varied conditions.
- Predictive Analytics. Algorithms learn from historical data to build various models. These models identify parts of a workflow that impact system performance. Enhanced predictive analysis will help predict potential usability issues before they occur. With predictive analytics, designers and developers can proactively address issues. Long before they become critical and cause system outages.
- Increased Fault and Bug Detection. Once a product is already deployed, any undetected Bugs become more costly and difficult to fix . AI algorithms can analyze auto-generated scripts to predict bugs. Bug-hunting algorithms learn from data, identifying correlations. This helps developers catch problems at the source and address and fix any defects.
- Shorter Development Times. Test automation speeds up the ‘Feedback Cycle’. Quick results provide a comprehensive understanding of user preferences and behaviors. They help companies iron out any kinks in their product. A quick turnaround helps them equip products and services to better meet user needs.
- Geographical Reach. In-person testing is geographically limited to small sample sizes. This makes it hard to gather diverse feedback. Remote user testing allows companies to reach participants way beyond ordinary reach. This encourages diverse and representative feedback. With AI translating languages, it removes the communication barrier. Researchers can now gather perspectives from different cultures. Remote testing is cost effective and convenient. It greatly reduces time and effort spent on user recruitment.
- Parallel Platform Testing. Cross-browser testing allows researchers to explore usability on different platforms. Companies test across operating systems, browsers, devices, screen resolutions and connection speeds. Manually testing the various platforms is tedious and time intensive.
- Test Script Reusability. Testing new devices involved creating distinct test scripts for each use case. The ability to re-use scripts saves a tester’s time and minimizes manual coding.
- Removes Barriers to Entry. Testing tools used to be prohibitively expensive and complicated. It made them inaccessible to small businesses and startups. This prevented many organizations from conducting any form of user testing. They deemed it too costly and specialized, and opted against them due to time constraints. With a sea of new tools, it offers small businesses a chance to scale up their design operations.
Automated user testing will help designers and researchers unearth meaningful insights. Powered by AI, UX professionals will use it to create products of exceptional quality.
Learn more about the pros and cons of AI in UX research.
Limitations of Automated User Testing with AI
Before diving headfirst in, we have some recommendations on how to use automated testing tools.
Establish where the machine’s involvement ends and human intervention begins. Here’s the other side of the coin — the limitations of using AI in automated testing:
- No Contextual Understanding. Accurate and meaningful insights need context. AI doesn’t ask for the study goals or prevailing research question. It also doesn’t have insight from previous research. We must mention that Marvin lets you search your entire research repository of older studies. AI testing systems don’t have background or contextual information about the study. They can’t comprehend the strategic reasons for carrying it out.
- Lack of Human Touch. Machines are NOT a replacement for researchers. They don’t possess human insight or user empathy. AI tools fail to grasp the subtle nuances and emotional depth from user feedback. Researchers must still design studies and bring context and human insight. They consider ethical and other factors, and get creative to solve problems. Humans must ALWAYS evaluate machine output.
- Accessibility Detection Issues. Some tools might struggle to address the complexities while testing for accessibility issues. The British Government conducted an experiment where they tested automated accessibility testing tools. They built a website loaded with accessibility issues and violations. Using 10 different tools, they audited the website for accessibility flaws. The most effective tool found only 40% of potential barriers on the webpage. A long way to go then.
- High Up-Front Cost. Again, we’re talking about the big 3: The initial time, resource and financial investment is significant. Testing software can cost big bucks. It also takes a significant amount of time and effort to set up. It may not always have everyone’s buy-in. Implementing new software is always met with resistance from employees. Change management is not to be overlooked. Companies must establish a champion of the cause.
- Unnecessary Data Capture. Systems running 24×7 capture large amounts of data. Some useful, most not. Parsing through often irrelevant data can be time consuming and overwhelming.
- AI’s Black Box. AI and ML applications use hundreds of algorithms to process data in a series of layers. The software returns an answer. However, researchers have no way of understanding how it got there. There’s no reliability in the results. Researchers have no way of validating results or tracing a claim or insight back to source.
Remember, automated testing with AI doesn’t replace manual testing. AI augments the user testing process. Companies will increasingly need skilled researchers. Smart testers who balance machine automation with human judgment. A tester’s role will become increasingly strategic. They’ll guide tests and make final decisions with the data available to them.
Setting up Automated Testing with AI
Craft an Automation Strategy
Here’s a step-by-step guide on how to set up a successful automation strategy:
- Define Goals. What do you want to achieve with testing? What do you want to learn? What type of data will you collect?
Tie user testing to business goals. Understand how projects will align with the company’s objectives. What KPIs will it affect?
Setting the right goals helps you choose the most appropriate testing method (and therefore tool). Without the ability to tie it back to strategic goals, it’s difficult to measure the ROI from a testing tool. - Establish Test Scenarios. To figure out the scope of automation, examine current processes. Look for areas that need optimizing. Decide what tests or test cases you will automate. Identify key user journeys and interactions that need automation. This enables targeted and thorough testing. Start with tests that require repetitive actions with vast amounts of data.
Choose tests that:
- Run for multiple builds, datasets, platforms or configurations
- Tend to cause human error
- Are time consuming
- Are impossible to perform manually
- Select the Right Tools. The answers to the first couple questions will dictate which type of tools you need. Do your research, conduct a comparative analysis (a researcher’s dream!) and choose a tool that fits your requirements. More in the section below.
- Review & Iterate. Continuously review the automated testing strategy. Keep adjusting the approach to ensure it meets your goals.
Setting up your user research strategy from scratch? Start here.
Desirable Features of AI Testing Tools
How will people interact with automation tools? Who will control use and reach across a company? It’s important to consider these factors when selecting the right automation tool:
- User Friendliness. How easy-to-use is the interface? Can non-UX professionals learn the ropes quickly?
- Research Capabilities. What research functionality do we need? Research, Recruit, Schedule or Testing? All of the above?
- Data Capture. Can the tool assimilate data from different sources? Video, Image, Interactions and Surveys?
- Reporting. Does the tool have reporting and visualization capabilities? Can I quickly see results and use that information?
- Integrations. Is the tool compatible with the company’s existing technology stack?
- Scalability. How will the product and business evolve? Can the tool handle the increasing needs of the research practice?
Learn how to use specific tools at each stage of the research process. Click here to head over to our guide.
Types of Testing Tools
The universe of automated testing tools spans far and wide. Each application within it handles a function, data type, or test type. We’ve compiled a list of functionalities to look out for…
[If you need a quick AI terminology refresher, head over to our glossary (of sorts). Thank us later.]
Test Plan Creation
Automated AI tools can expedite many aspects of planning a study. Use generative tools to create outlines and scripts for research. AI tools help during Lit Reviews. They scan pdfs and summarize long bodies of texts to extract meaningful information. Before you begin crafting interview discussion guides, use a tool to generate questionnaires. Feed questions back into the tool to fine-tune them and eliminate bias from your study.
Content Generation
Create design prototypes and visual content for user interfaces. Tools such as Uizard allow you to create several wireframes and test each option. Specify user requirements and design principles and let the tools do the rest. Text-to-Image (and other) tools rely on specific user input. Use Dall E-2 and Midjourney to create stunning images and videos from a single prompt.
Creating the perfect prompt is an iterative process. Check out Marvin’s tips and tricks for mastering AI.
UX Writing falls under the umbrella of content generation. However, it warrants its own mention. AI-powered tools vastly improve the speed and quality of writing. They are a limitless source of fresh content. Tools can eliminate grammatical errors and suggest language improvements. They help maintain a consistent tone of voice and optimize content for SEO. Tailor content to specific user groups and even translate content into different languages. Use generative AI to create a multiple content options for A/B testing.
User Personas
User persona software helps designers create well rounded customer profiles. Researchers create cards that capture demographic and psychographic information of users. They help define a user’s goals, attitudes, motivations, needs and pain points. Generate user stories and journeys. Chart different users’ navigation in order to prioritize design elements.
A quick word on synthetic users — they don’t replace real users (and never will). Companies can, however, simulate user interactions and check tests for real user data. It opens the door for small companies to conduct testing with statistical significance.
Automated Design
Tools can expedite various routine design functions to make a designer’s life easier. Tinker with visual elements using the following software:
- Vectorizers convert any illustration to a high quality vector image.
- Font tools recommend pairings that enhance a copy’s readability and visual appeal.
- Color Palette tools suggest colors that mesh well together in line with brand colors. Use these tools to create consistency across designs
- Logo Makers perform exactly as advertised.
Participant Recruitment
Finding and managing participants for research studies is cumbersome. Our friends at User Interviews (and similar tools) help streamline the process. Tools tap into a large and diverse participant pool, screening and matching participants with studies. This generates high quality recruitment. Tools now facilitate recruitment, interview scheduling and incentive distribution all in one place. Complying with data protection laws is essential. It ensures they not only protect participants’ information, but also the research itself.
Usability Testing
These tools track users’ behavior. Researchers conduct an in-depth assessment as users navigate through a product or application. Test results offer actionable insights. It helps designers iterate and enhance the user experience in products and services. Usability testing tools are not location dependent. They enable testing in real-world contexts, environments the product is designed for. Tools leverage remote user testing to assemble diverse feedback.
Which users hit their goals and who struggled? The following analysis helps determine what areas designers should focus on:
- Attention Analysis. Use attention analysis to identify a user’s focus on visual assets. Eye tracking and heatmap technology track where users look on a screen (and how long). This provides important insights into where people concentrate their attention.
- A/B Testing. Compares two or more variations of a design element to learn which performs better. This includes layouts, visual elements and copy. These tools offer insight into user preferences using quantitative metrics. Designers use this feedback to implement layout changes and improve site navigation.
- Card Sorting & Tree Testing. These tools improve information architecture of websites and applications. Card sorting involves users explaining their mental models and how they categorize information. Tree Testing evaluates the effectiveness of navigation. It puts users to the test, asking them to carry out tasks and find information. Both offer a way to align information architecture and navigation with user expectations.
Sentiment Analysis
Help researchers understand user opinions, emotions and attitudes when interacting with a product. Algorithms analyze text responses from users. They classify phrases based on emotion of each word – positive, neutral or negative. AI can blitz through transcripts, social media posts, forums and customer feedback. For advanced emotion recognition, some tools offer speech and facial analysis software. With it, they understand more about a user’s emotions as they navigate through a product. Sentiment analysis tools help build more empathetic user experiences. Ones tailored to different users or user groups.
Surveys and User Interviews
These tools facilitate the collection and analysis of user feedback. Create and distribute customizable surveys with multiple question types. Survey tools are now equipped with robust reporting and data analysis capabilities. (And many qualitative data analysis tools are now equipped with survey capabilities!)
Analyze behavior in real-time. Ask users questions as they navigate through interfaces for in-context feedback. Record sessions so you can refer to them later during analysis and synthesis.
Human moderators are no longer needed to guide participants through the session. AI can convert text to voice overs and incorporate them into AI avatars. This helps save time and money by eliminating human moderators.
Documentation
Increase the efficiency and effectiveness of qualitative data collection. AI generates verbatim transcripts so you can concentrate wholly on users during interviews. It can sift through lengthy transcripts to create text summaries. Some tools offer time-stamped AI notes and highlights of user feedback and experiences. This not only saves the researcher time. It gives them a foundational layer of analysis before they begin dissecting interviews.
Once collected, all your data needs a place to live. A searchable, centralized repository will do nicely. One that’s accessible to many teams involved in the product roadmap. A tool that integrates seamlessly with existing software.
Kill two birds with one stone — see why our clients call Marvin a game changer.

Best Practices for Integrating AI Testing into UX Research
Ensure that your automation strategy delivers the best return on investment (ROI). Integrate the following practices to create efficiencies in your UX process:
- Create a Well-Defined Testing Strategy. A usability test plan acts as a roadmap that lays out all the steps for user testing. Design a comprehensive test plan and execution strategy. One that encompasses various scenarios, user journeys and anticipated interactions.
- Divide Tasks. Assign people tasks to perform (automated testing will always need supervision!) based on an individual’s skill set and expertise.
- Collaborate. Engage with in-house teams. Share the tool and its benefits with designers, developers and testers. Optimize the internal flow of information. Gathering feedback and validating ideas quickly before beginning design work is essential. Establish collective ownership so everyone feels responsible and accountable. Internal teams will be able to connect with end users at all developmental stages. It’ll also help everyone get on board the automated AI train. Full steam ahead!
- Test on Real Devices. No simulator or emulator can replicate real user conditions. Experiences must be seamless no matter the device or platform. Applications need to work in real-life situations (e.g., low battery or spotty network areas). Companies who don’t test on real devices suffer. They run the risk of bugs in deployed apps. All leading to some very unhappy users.
- Record Your Work. Keep detailed records of previously conducted tests. Future testers will be able to identify reasons for failure or success. These logs help in debugging.
- Continuously Monitor and Learn. App testing isn’t just a one-off. It must be a continuous process. Analyze the digital experience over time. Learn from data and look out for trends that inform decision making. Regularly validate and optimize testing scripts to reflect changing user behaviors. Keep abreast with AI tech updates for new features and functionality.
We share some tips on how to master user research software.
Common Pitfalls and How to Avoid Them
Below are some major faux-pas for researchers and designers to steer clear of.
While using testing tools, DO NOT:
- Neglect Data Privacy. Ensure robust data management practices are in place. Companies must handle users’ personally identifiable information (PII) carefully. Safeguarding this data establishes user trust. Tools of choice must anonymize user information and comply with data protection regulations. With Marvin, your user data is always protected.
- Treat AI results as the final word. Researchers must carefully review and interpret results. A human must check whether they are valid, accurate and useful. Beware of automation bias. Review and validate AI’s results. Cross reference them with qualitative source data. This ensures they are free from bias and misrepresentation.
- Roll out company wide. Yet. Start small with your testing efforts. We recommend conducting a soft launch. Use data that you’ve already collected and analyzed. You’ll be able to observe whether the tool is missing important items before its full launch. Train your AI — provide diverse training data so it can learn, adapt and refine its learning process. Make sure it isn’t biased though!
Real-World Implementation
Companies are adapting their research process to incorporate automated testing. They’re transitioning from manual testing to a hybrid approach.
And they’re reaping the benefits.
Large tech companies have successfully implemented automated user testing:
- Netflix uses automated A/B testing. It helps them optimize user interfaces and personalize content recommendations.
- Google uses TensorFlow for automated image recognition in UX research.
- Facebook updates their app regularly. When they push a change or update their app, they conduct automated testing. Usability tests examine whether product updates affect the user experience.
- Amazon conducts automated testing behind the scenes. They search for bugs on their platform. It’s used to maintain uniformity in the user experience across different devices.
- Microsoft uses automation to ensure its various products maintain compatibility with one another. It ensures that applications work consistently across all platforms.
The Future of Automated User Testing
Automated user testing saves company resources. Businesses benefit from real-time insights. On an ongoing basis, testing helps development teams fix bugs before they happen. This speeds up the design process and boosts data-led decision making.
Automated testing technologies of the future will continue to advance in their functionality. ML will eventually act as a facilitator to deliver end-to-end research automation. Systems will continuously learn from data and recommend what tests to conduct. They’ll predict the impact on businesses.
AI augments research and design work by performing the heavy lifting. This allows researchers and designers to concentrate on more strategic pursuits.
It all points to richer experiences and more personalized user interfaces.
A future with automated testing is here already. Designers and researchers must begin integrating automated user testing into their UX research.
Photo by Lugman .S on Unsplash (with some minor edits for color)