Survey data should be a goldmine of insights.
But when you first dive in, it’s more of a swamp. You’ve got heaps of ratings, open-ended responses, and even a few head-scratching comments.
The trick to making sense of it all? Smart analysis.
This guide will walk you through the best survey analysis methods, tools, and tips to turn your raw data into clear, actionable insights.
And when you’re ready to cut through the clutter, our AI-driven research assistant is here to help. Sign up for a free Marvin account, and start uncovering survey insights with speed, depth, and clarity.

What is Survey Analysis?
Survey results analysis is the thrilling (and often panic-inducing) process of interpreting hundreds or thousands of survey responses to uncover patterns, insights, and trends.
You break down quantitative data (such as ratings and response counts) and qualitative data (the gems in open-ended responses), looking for connections that show the big picture — what users think, need, or wish would change.
Those Aha! moments when you spot the underlying themes in your survey responses are priceless. But the road to it is paved with survey analysis. Every answer is a clue you piece together to map out trends and user pain points.
Essentially, survey analysis translates raw feedback into actionable insights using the following operations:
- Categorize answers to find recurring themes
- Calculate averages to see the general satisfaction levels or trends
- Identify correlations that reveal relationships between user needs and behaviors
Survey Analysis Example
In the context of evaluating a new app feature, you might survey users on usability, visual appeal, and functionality.
Survey analysis could reveal that users aged 25-34 find navigation challenging, with comments highlighting “hard-to-find options.”
This insight points to a design flaw, guiding your team to refine navigation for better usability.
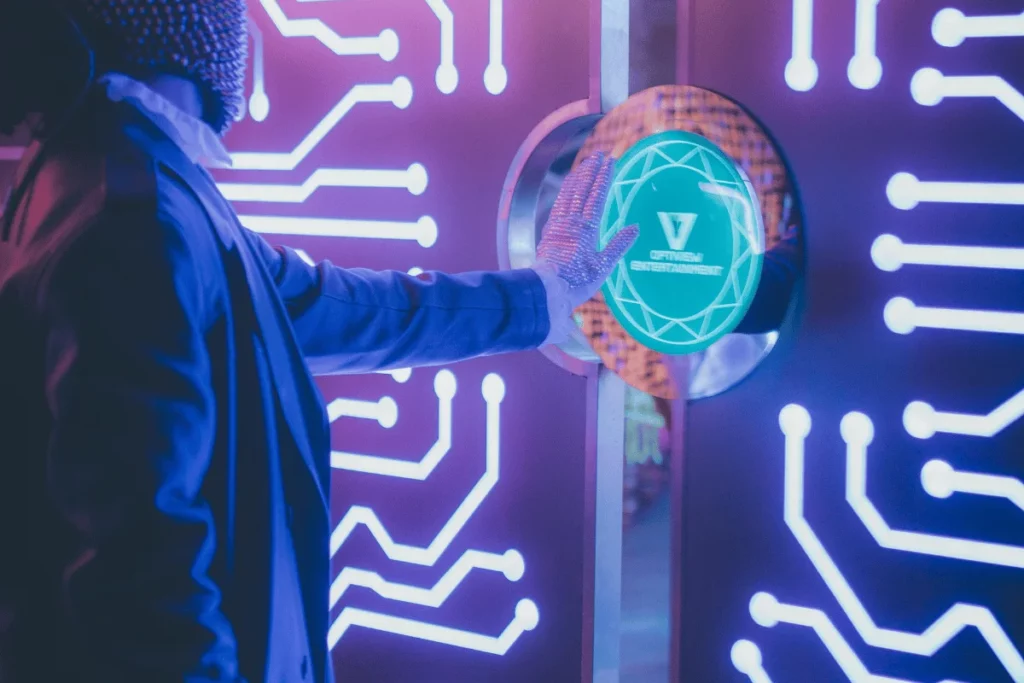
Quantitative Survey Analysis Methods
The survey analysis methods that focus on quantitative data give structure to numbers, turning them into digestible insights.
These techniques help you uncover trends, validate assumptions, and pinpoint relationships.
Below is your toolkit for making sense of quantitative survey data.
Descriptive Statistics
Using averages, medians, and frequencies, descriptive statistics give you a quick snapshot of usage patterns.
For example, you could survey users on how often they use a specific app feature, from “daily” to “never.” The results will reveal the most common frequency, helping you understand if a feature is popular or ignored.
Inferential Statistics
With inferential statistics, you can use one sample to make predictions about a larger population.
If you surveyed only 500 users about an app update and 80% are satisfied, you can predict that most users will likely feel the same.
This analysis method allows you to scale insights without surveying everyone and their cousin.
Cross-Tabulation Analysis
Cross-tabs compare responses across different user groups, revealing patterns and preferences.
For example, you surveyed users on feature preferences by age. Cross-tabulation could reveal that users aged 18-24 favor simple layouts, while 35+ prefer advanced options.
In short, this analysis shows who likes what. It helps you tailor future design decisions without making assumptions about what users expect.
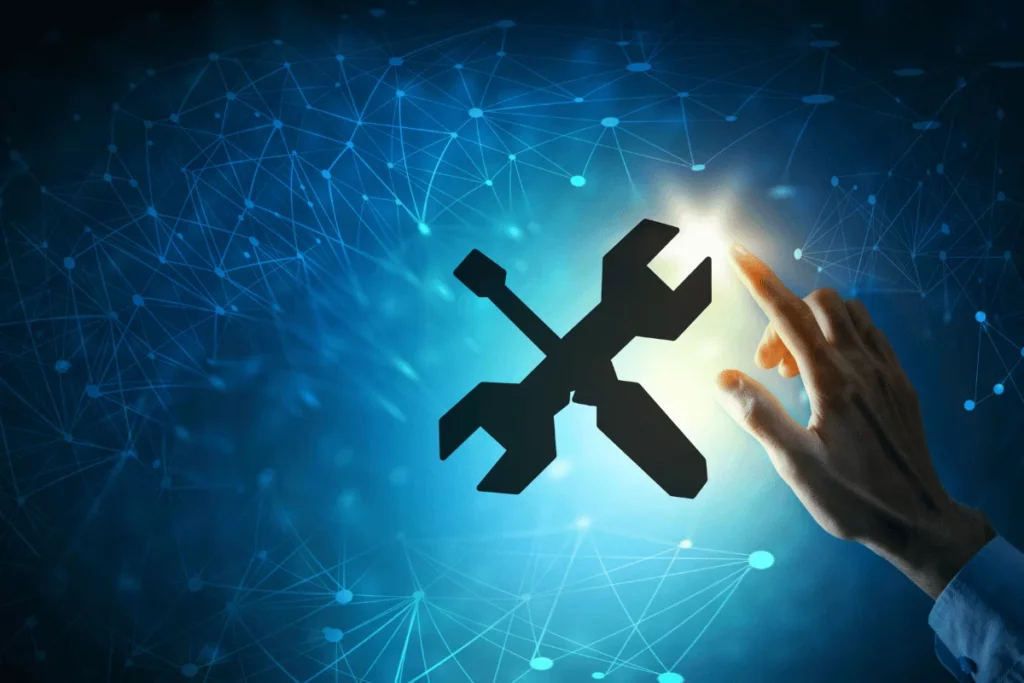
Regression Analysis
Through regression analysis, you can identify relationships between variables and understand how they affect each other.
One use case is testing if users who spend longer in your app report higher satisfaction.
With this method, you can confirm if there’s a connection between time spent in the app and user satisfaction. Based on the results, you’ll guide design tweaks that could boost engagement and happiness.
The T-Test
This survey analysis method is very similar to A/B testing in marketing. The T-test compares means between two groups to determine if the differences are significant.
Imagine you launched two versions of an app feature and surveyed user satisfaction with each. The T-test can reveal if one version outperforms the other by far, removing the guesswork from your decision-making.
Conjoint Analysis
The conjoint analysis simulates choices to help you assess which feature combinations users value most.
For example, when designing a productivity app, you can test combinations such as “fast loading,” “custom themes,” and “offline access.”
A conjoint analysis survey might reveal that users prioritize “fast loading” with “offline access” over aesthetic options.
This insight guides your team to focus on the features users find most essential, leading to a design that resonates with their needs.
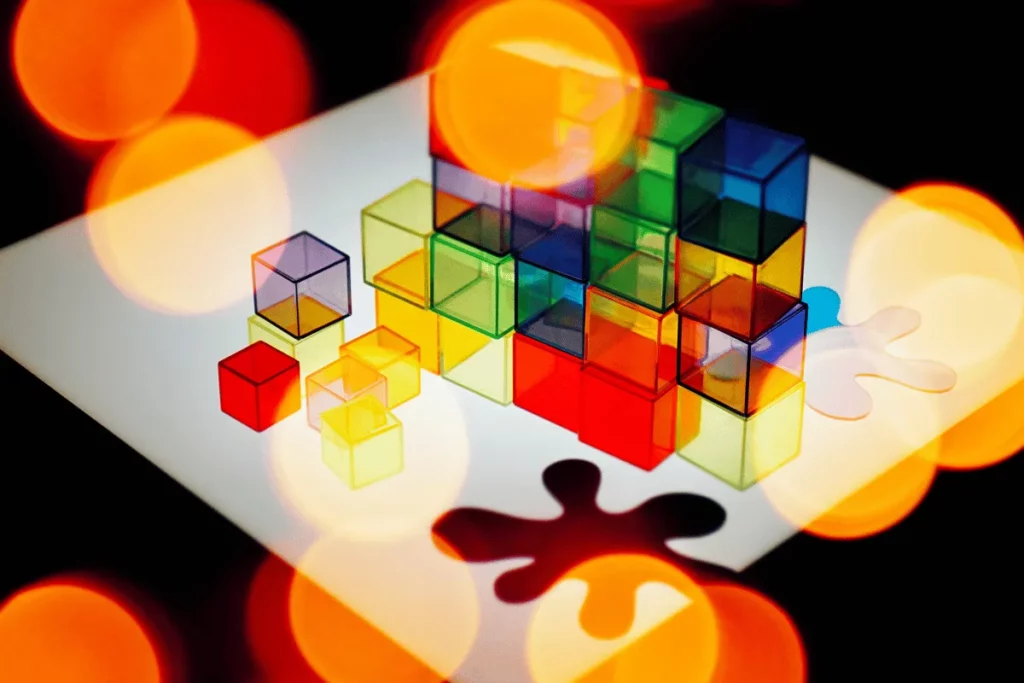
Qualitative Survey Analysis Methods
The survey analysis methods that focus on qualitative data explore the stories, themes, and rants in open-ended responses.
With these techniques, you can uncover the “why” behind the data and understand users’ emotions, beliefs, and preferences.
Here’s how you can distill meaning from qualitative survey responses.
Thematic Analysis
Searching for recurring issues or sentiments? Thematic analysis is the method you want to use. It’s perfect for identifying common themes and will help you group similar ideas to uncover patterns.
In a survey on app usability, thematic analysis could reveal that themes such as “ease of navigation” and “loading speed” keep popping up.
By recognizing these themes, you can see what users value most and suggest app improvements where they’ll make the biggest impact.
Content Analysis
One way to reduce words to their essence and turn them into measurable insights is through content analysis. This method codes qualitative data into categories and quantifies them.
For instance, if users provide feedback on app features, you can categorize responses as “positive,” “negative,” or “neutral.”
From here, you calculate how many users fall into each category and get the big-picture view of overall sentiment. When you look at user opinion frequency, it’s obvious which areas need improvement.
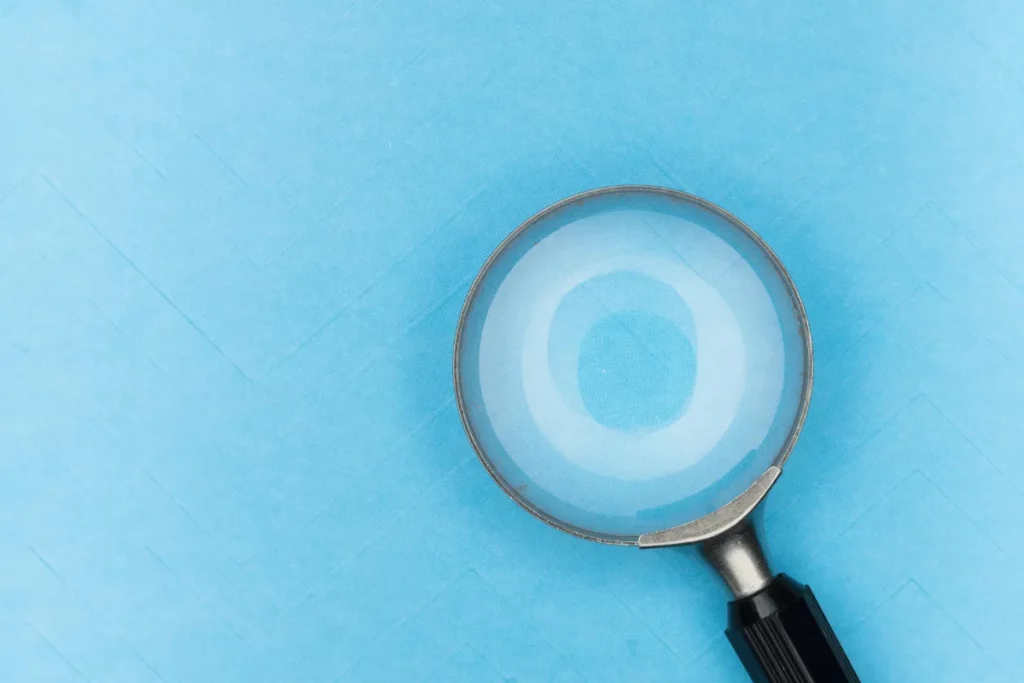
Narrative Analysis
Narrative analysis dissects individual stories to understand user experiences and perspectives. It gives context to user feedback, helping you improve experiences based on real-life scenarios.
This method is particularly beneficial when you study personal user journeys. For example, analyzing a user’s story about onboarding might reveal feelings of overwhelm from too many setup steps, struggles with finding resources, or difficulties navigating certain features.
A quantitative analysis method applied to the same experience might only yield an average satisfaction rating for onboarding. In any case, it would lack the nuanced emotional responses and specific pain points that narrative analysis uncovers.
Grounded Theory
Unlike traditional approaches that start with a hypothesis, grounded theory lets insights come out of user data.
You dive into survey responses, staying open to unexpected themes that surface organically. As you analyze, recurring patterns begin to pop up across responses. Those patterns will guide your decisions more confidently because they’re grounded in real user data.
Think about analyzing open-ended feedback and finding that the “frustration with login processes” theme keeps surfacing. With grounded theory, you can conclude that you need to simplify user access.
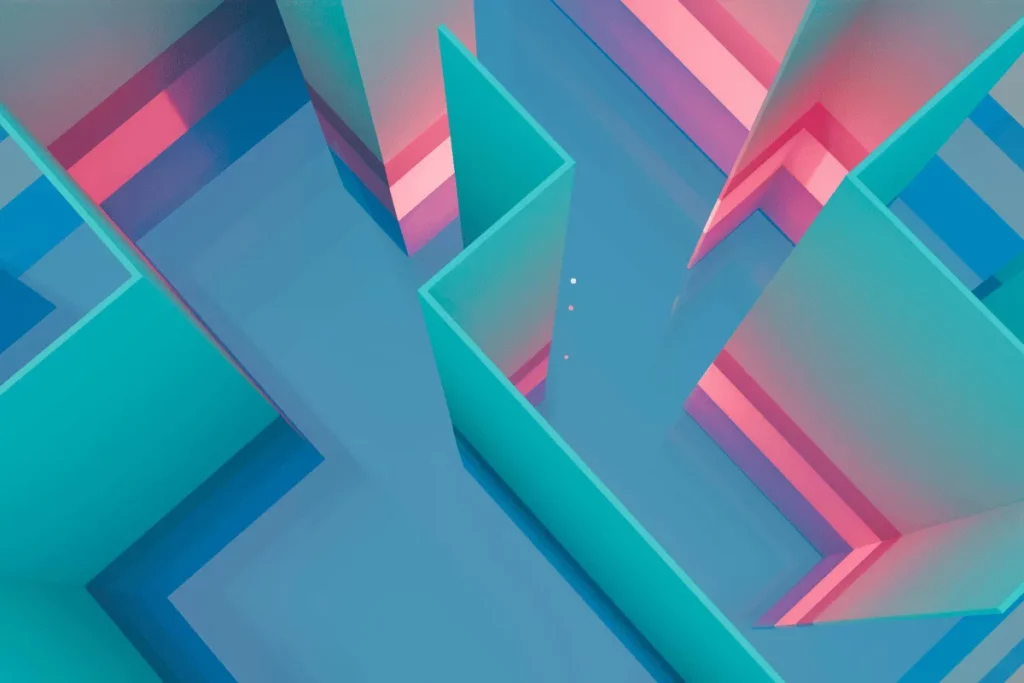
Mixed Methods Survey Approach
The mixed approach combines quantitative and qualitative techniques to capture both the “what” and the “why.”
By doing so, you understand how users respond and their motivations, taking user-centered decisions with a dash of certainty and empathy — something you can’t get from ratings, counts, and averages alone.
Here’s how this might work when assessing an app dashboard redesign.
You look at the quantitative data and notice users log in 40% more after the redesign, suggesting they like it.
However, qualitative feedback reveals a crucial insight: users appreciate the new tracking features but are frustrated with the placement of navigation buttons.
With this mixed-methods approach, you confirm that there are successful elements you can keep, but you also need to address pain points. That’s how you get to a more effective, user-centered redesign.
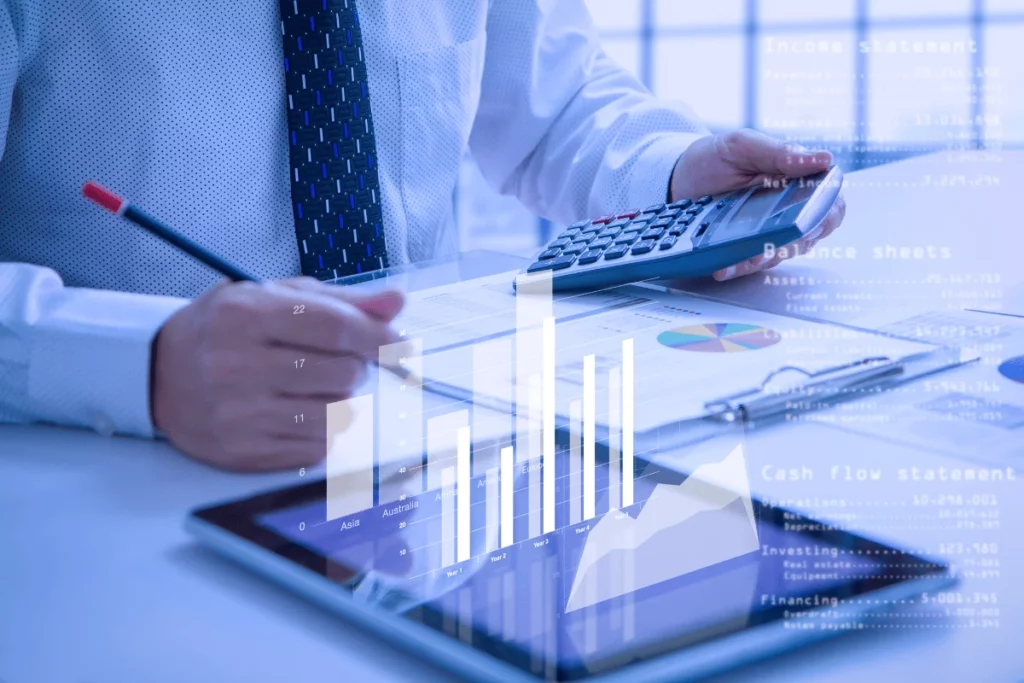
Best Survey Analysis Software
The best survey analysis tools can turn massive amounts of responses into clear, actionable insights. Here are four top software picks that can help make your analysis easier, faster, and more insightful:
Tools | Features | Benefits | Pricing |
Marvin | * Automated NPS analysis * Correlation identification between responses * Excel data analysis * Charts and graphs generation * Enhanced data security * Ask AI on survey data | * Has packages for all team sizes * Uses advanced AI to automate analysis * Quickly processes thousands of responses * Presents insights in easy-to-understand visuals | * Free with limited functionalities * $50/user/mo (Essentials) * $100/user/mo (Standard) * Custom (Enterprise) |
Qualtrics | * Advanced analytics and visualization tools * Customizable reporting options * Integration with other AI research tools * Survey distribution across multiple channels | * Flexible for complex analysis needs * Works well for medium to large teams * Provides in-depth, customizable reports | * Available on demand |
SurveyMonkey | * Instant graph and chart generation * Trend identification * Customizable survey templates * Automated reporting | * Easy to use, especially by small teams * Fast visualization of survey data * Requires no complex setup | * Individual plans for $39 and $99 a month * Team plans for at least 3 users for $30 and $75/user/month |
QuestionPro | * Survey templates and customizable question types * Survey distribution across email, social media, and mobile apps * Basic data visualization tools and sentiment analysis * API integration for connecting with other data platforms | * Quick setup for feedback collection from various channels * Real-time reporting with easy-to-read visualizations * Good for large-scale survey distribution and response management | * Free with limited capabilities * $99/user/mo (Advanced) * $83/user/mo (Team edition) * Custom (Research edition) |
While all four survey analysis software offer solid features, Marvin is the standout choice. Its AI-driven analysis quickly processes thousands of responses, helping you make sense of both closed-ended and open-ended answers effortlessly.
Plus, Marvin’s flexible pricing packages accommodate projects of all sizes, whether you’re running small surveys or managing complex, large-scale research.
Sign up for a free account and experience Marvin’s powerful analysis firsthand!
Best Practices for Survey Data Analysis
Even with the best-crafted survey questions, the responses can seem overwhelming. Staring at that giant pile of information (instead of the structured insights you hoped for) is intimidating. But the following best practices will help you make sense of it all:
- Clean your data: Remove incomplete or random responses to keep results accurate.
- Segment wisely: Break down responses by demographics to spot unique trends across groups.
- Use visualizations: Charts and graphs make insights clearer and bring data to life.
- Focus on key metrics: Stick to metrics that align with your survey goals.
- Double-check insights: Look for patterns to ensure your conclusions hold up.
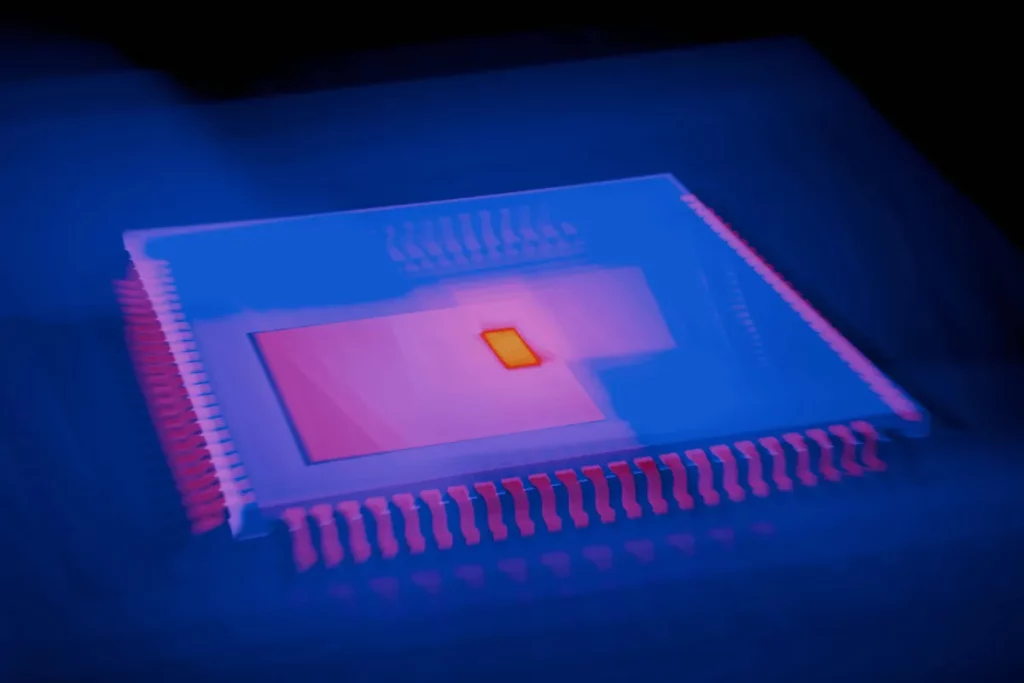
Frequently Asked Questions (FAQs)
Time to answer some of your open-ended questions on survey analysis methods:
How Can I Ensure the Reliability of My Survey Data?
When formulating your survey, stay consistent in question-wording and avoid leading questions. Test your questionnaire with a few persons before blasting it to everyone. And as you start gathering responses, clean your data to remove incomplete or biased answers.
What Are the Limitations of Survey Analysis?
Survey analysis can show you trends but often lacks the “why” behind them. Its most significant limitations are response biases and the inability to capture deep, contextual insights from closed-ended questions.
Does Survey Length Affect Response Quality?
Yes! Long surveys can lead to survey fatigue, where respondents lose focus or drop out. Aim for brevity and keep questions relevant to maintain higher response quality and engagement.

Conclusion
Analysis of survey data doesn’t have to be an overwhelming process.
By following best practices and leveraging the best survey analysis tool, you’ll turn raw feedback into decisions that resonate with users.
For a faster, smarter way to get there, Marvin is ready to be your ultimate research repository and AI assistant.Discover how Marvin can simplify your survey analysis — book a demo today!