Qualitative data is jam-packed with the “Aha!” and “Huh?!” moments that make or break the user experience.
Any passionate researcher values this complex information.
Leaders, however, want to see measurable trends and data to make confident decisions. Getting stakeholder buy-in is much easier when you present your findings in a numeric, trackable, and visually compelling format.
In case you’re wondering, “Isn’t quantification going to lose the richness of my data?” the answer is, “Not if you do it right.” And that’s what you’ll learn in this blog post with:
- A full guide on how to quantify qualitative data without oversimplifying your research findings
- An introduction to Marvin, our AI-powered qualitative data analysis platform
Create your free account with Marvin to centralize, analyze, and visualize user feedback into insights that stakeholders can instantly grasp.

What is Qualitative Data?
Qualitative data captures the user feedback that numbers can’t — the thoughts, experiences, and gut reactions people have with your product. It gives you the “why” behind user actions.
For example, instead of asking users to rate your new interface from 1 to 5 (that’s the quantitative approach), you’d ask, “What do you think of our new interface?”
Responses such as “The menu feels clunky” or “This workflow confuses me” are your qualitative data in action.
When and Why Qualitative Data Is Valuable?
In early design or development, qualitative data reveals user expectations and preferences. Later, it uncovers usability issues or emotional reactions that metrics can’t show.
Qualitative observation is valuable when you need context, meaning, and depth. It provides the “human” layer for designing functional solutions that genuinely connect with users. It’s especially useful for the following purposes:
- Refining products
- Exploring new concepts
- Identifying unexpected pain points
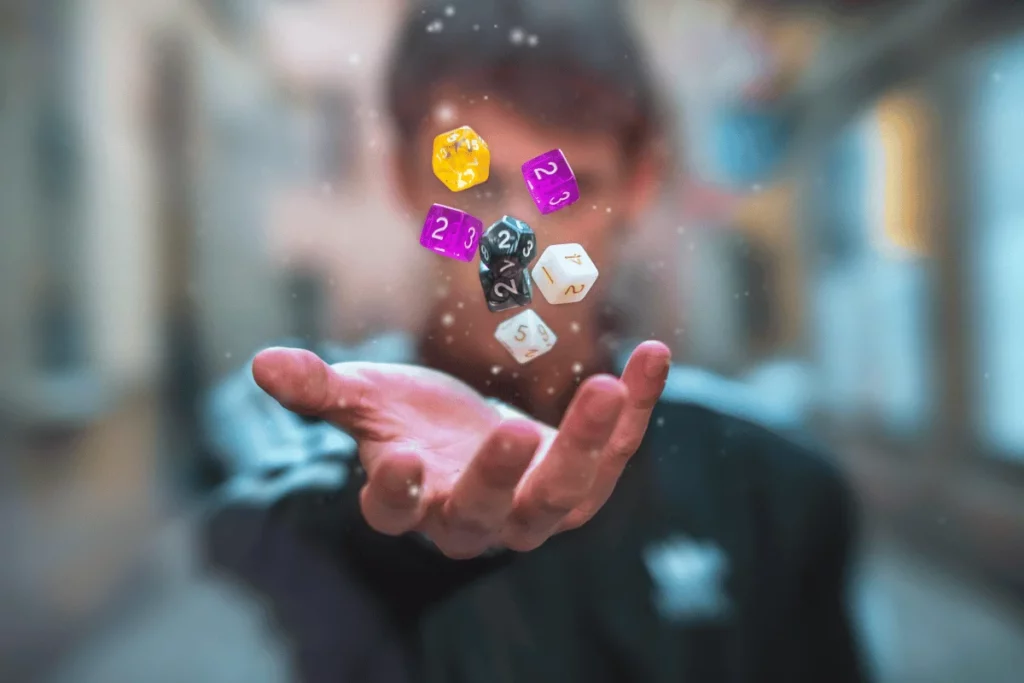
What Does It Mean to Quantify Data?
When quantifying data, you turn raw, open-ended feedback into measurable insights. It’s a process of identifying patterns and assigning values or categories to see how often they occur.
For instance, you may want to quantify user comments about a new feature. You notice “Some users couldn’t find the feature in the menus” by simply reading their answers.
With quantification, however, you can say, “60 percent of users felt the feature was hard to find in the menus.” This formulation shows a significant number of users had this issue, so you can prioritize fixing it.
Benefits of Quantifying Qualitative Data
Quantification lets you keep all the depth of qualitative data in a format you can prioritize and measure over time. Here are some key benefits:
- Spot patterns: Quickly see what issues your users are facing, such as hard-to-find buttons or confusing flows.
- Prioritize fixes: Focus on what impacts the most users and solve the high-impact problems first.
- Communicate clearly: Give your team data-backed insights, not just general observations.
- Track progress: Use numbers instead of gut feelings to measure if your changes improve things.
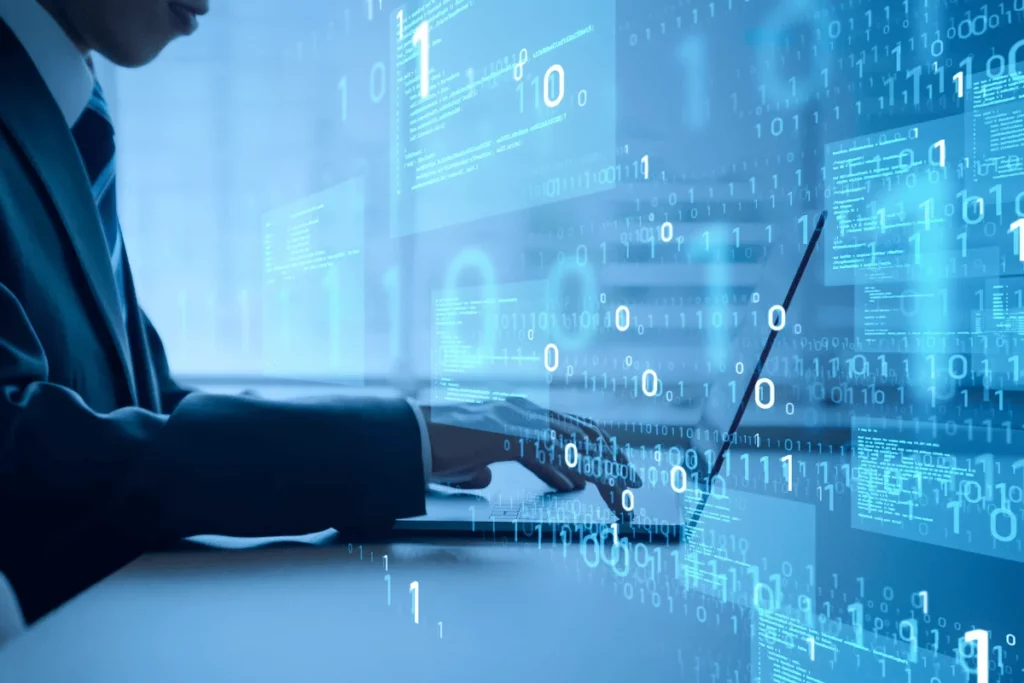
How to Quantify Qualitative Data
Let’s break down how to quantify qualitative data clearly and effectively.
Define Your Goals
Start by knowing what you’re after.
Are you trying to understand why users get stuck in a certain flow? Or maybe you want to measure overall satisfaction with a new feature?
Clear goals narrow your focus and help you stay on track.
Organize Your Data
Next, gather your feedback and group it by themes.
For instance, if users are giving feedback on your new app layout, group comments about “navigation,” “buttons,” or “color scheme.”
This way, you’re not sifting through scattered feedback. You’re looking at organized, structured themes.
Choose Your Method(s)
Now, it’s time to decide how you’ll tackle the data.
Quantifying qualitative data often requires multiple methods to get a full picture.
You might choose sentiment analysis to measure user emotions and then apply coding to sort feedback into specific categories.
Set Up Clear Categories or Codes
This step is what turns qualitative insights into measurable, trackable data points.
Create specific categories that match your goals. These should cover the main topics you’ve found, such as “ease of use,” “visual appeal,” or “loading speed.”
Keep each category distinct so every piece of feedback has a clear home.
Count the Responses
Now, it’s time to get counting. Go through each category and tally up how often each appears.
For example, if “ease of use” comes up in 30 percent of feedback, that tells you it’s a top priority for users.
These counts let you see which themes need the most attention and give you a way to measure changes over time.
Review and Adjust
This last step ensures you’re not leaving anything out and your data accurately reflects user insights.
Make sure all feedback is in the correct category and aligns with the structure of your analysis.
If something doesn’t fit, consider adding a new category or refining existing ones.
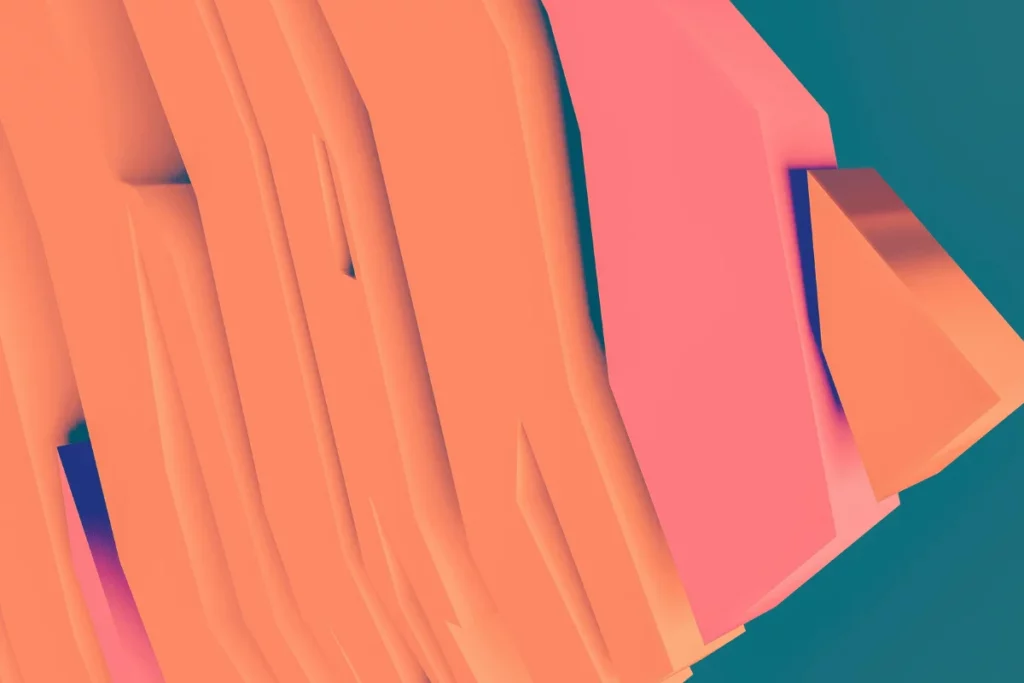
5 Methods to Quantify Qualitative Data
Quantifying qualitative data is more manageable when you know where and what to look for. Below, we summarize five essential methods:
- Content analysis
- Sentiment analysis
- NPS analysis
- Thematic analysis
- Keyword frequency analysis
Our AI-powered research assistant supports all of these. Sign up with Marvin today for free to speed up your analysis (and organize it with canvas and Kanban boards).
1. Content Analysis
When you analyze content, you tag specific words, phrases, or topics.
Whether it’s a long, messy interview transcript, hundreds of customer reviews, or a pile of open-ended survey responses, you’re turning it into structured information.
This method lets you spot recurring topics or concerns across user feedback.
Content Analysis Tools
NVivo and MAXQDA can run content analysis. They let you tag and categorize text and generate word clouds or frequency graphs to spot patterns.
Content Analysis Example
Your feedback analysis reveals users frequently mention “load time.” By tagging each mention, you quantify that 35 percent bring it up. That’s clear evidence to prioritize load time improvements in your next product sprint.
2. Sentiment Analysis
This approach moves beyond what users say and explores the emotions behind their words. You’re measuring the emotional tone to understand if they feel positive, negative, or neutral.
Sentiment Analysis Tools
MonkeyLearn and IBM Watson use AI to assess sentiment and tag responses with emotional values. They can capture user mood and provide an overall view of satisfaction across feedback.
Sentiment Analysis Example
You’ve launched a new feature, and user reviews are mixed. Sentiment analysis indicates that 70% of responses are positive, while 30% are negative.
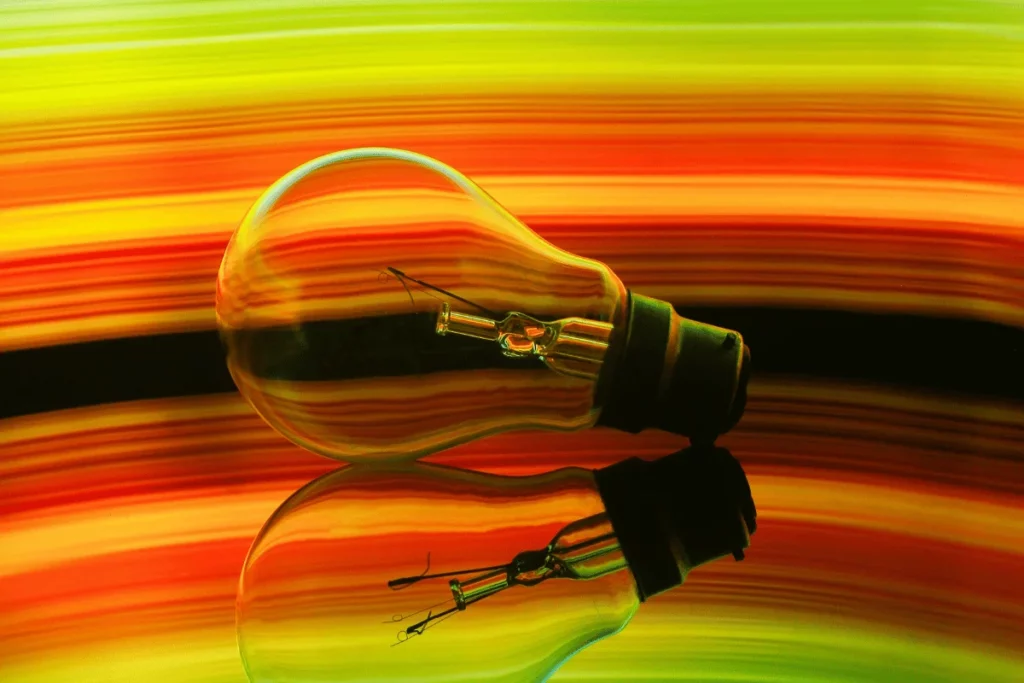
3. NPS Analysis
NPS (Net Promoter Score) measures customer loyalty by asking, “How likely are you to recommend this product?”
Responses fall into three categories:
- Promoters: 9-10
- Passives: 7-8
- Detractors: 0-6
Subtracting the percentage of detractors from promoters gives you an NPS score from -100 to +100.
Unlike sentiment analysis, which captures emotions, NPS focuses on loyalty. The open-ended follow-up question, “Why did you give this score?” adds a valuable layer of qualitative feedback to explain their loyalty.
NPS Analysis Tools
Marvin makes NPS analysis both powerful and effortless. You import customer surveys into the platform and let the AI uncover insights about your promoters and detractors.
With our AI research assistant, you can even find correlations between responses.
NPS Analysis Example
You’ve launched a new feature that earns a solid NPS of +30, with 60% promoters, 20% passives, and 20% detractors.
By analyzing the feedback, you notice promoters love the ease of use, while detractors are frustrated by load times. This insight guides you in optimizing load times and converting detractors into promoters.
4. Thematic Analysis
The thematic analysis looks at the recurring themes in your qualitative data. It doesn’t just count words but drills down to the ideas users keep mentioning.
Grouping feedback into themes gives you a clear picture of what matters most to users.
Thematic Analysis Tools
Harnessing the power of AI, Marvin quantifies your qualitative data and automatically groups it by key themes.
Other tools you can test to tag, organize, and visualize data into themes are Atlas.ti and Dedoose.
Thematic Analysis Example
Users leave feedback on your app’s new navigation feature. Through thematic analysis, you identify how they feel regarding topics such as “ease of use,” “accessibility,” and “visual appeal.”
Content Analysis vs. Thematic Analysis
While content analysis is about counting specific words or phrases, thematic analysis looks for their meaning. Here’s a quick side-by-side comparison:
Aspect | Content Analysis | Thematic Analysis |
Purpose | Quantify word/phrase frequency | Identify underlying themes or ideas |
Focus | Specific terms or keywords | Broader patterns and concepts |
Output | Data on most common words/phrases | Rich themes that provide deeper context |
Ideal for | Spotting high-frequency issues | Understanding complex user experiences |
5. Narrative Analysis
Focusing on how users describe their experiences, the narrative analysis examines the structure and flow of user stories. The goal is to help you understand the following:
- What users say
- How they share their journey
- What are their motivations, values, and perceptions of your product
Narrative Analysis Tools
NVivo and Dedoose can also handle narrative analysis. They let you tag and organize stories within your data. And they capture story arcs and recurring themes to see how user journeys change.
Narrative Analysis Example
Users describe their onboarding experience with your app. Narrative analysis reveals common story elements such as initial confusion, gradual learning, and eventual success.
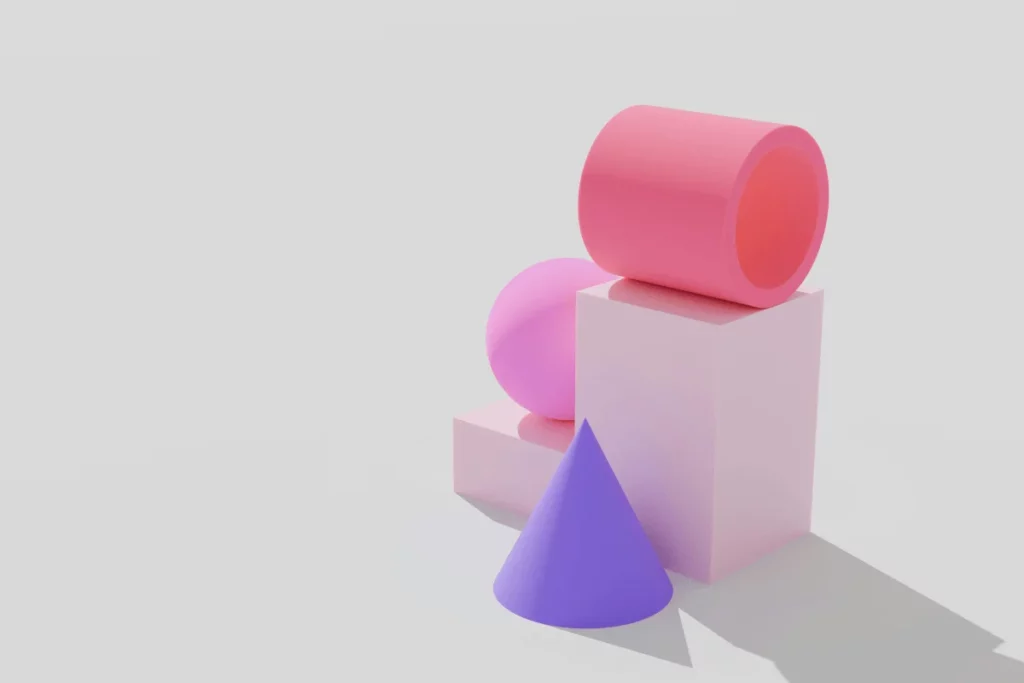
Quantifying Customer Feedback
The methods above focus on what to analyze in qualitative data: themes, sentiments, satisfaction, the customer journey, etc.
Now, let’s look at how to do it — the practical steps to categorize, count, and code your qualitative data:
- Coding and Tagging: Assign themes or categories (such as “usability” or “performance”) to structure feedback. Marvin’s automated features have your back here, saving time and boosting accuracy.
- Binary Coding: For targeted feedback, such as whether users struggled to find a feature, use a simple yes/no structure. You’ll remove the guesswork and pinpoint issues across large datasets.
- Scale-Based Quantification: With nuanced feedback, assign a score (1-5) based on intensity or positivity. When a user comments, “Navigation is a bit clunky but manageable,” that could be a solid 3 on usability.
- Keyword Frequency Analysis: Count how often specific keywords pop up. Marvin’s tagging hierarchy makes this quick, showing you top user issues. If “load time” keeps surfacing, you’ll know it’s a hot topic.
- Sentiment Quantification: Use sentiment analysis to capture user mood on a positive-to-negative scale. This lets you keep tabs on overall satisfaction, helping you track if user sentiment shifts after product updates.
- NPS-Linked Thematic Analysis: Dive into themes within each NPS group (Promoters, Passives, Detractors) to see what drives loyalty (or lack of it). If Detractors keep mentioning “response time,” you’ve got a clear signal on where to make changes to win them over.
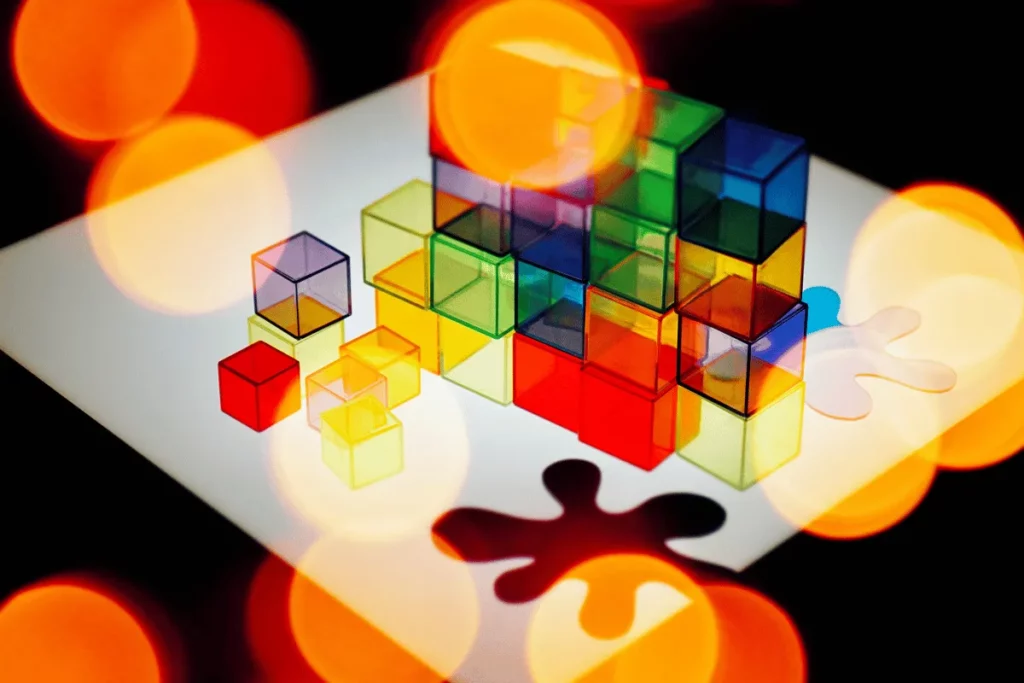
Challenges in Quantifying Qualitative Data
With rich, detailed feedback come some tricky obstacles. Here are a few challenges to keep in mind:
- Losing nuance: Turning words into numbers can oversimplify complex user feedback, losing valuable context.
- Inconsistent language: Users describe things differently, making it harder to classify feedback cleanly.
- Bias in coding: Personal interpretation can sneak into coding, affecting the objectivity of your results.
- Overlapping themes: Feedback often spans multiple themes, making it tough to fit neatly into one category.
- Time-intensive: Manual coding and tagging take time (especially with large datasets), which can slow down analysis.
Pro Tip: Triangulation in qualitative research can help address these potential issues by cross-verifying results with multiple methods.
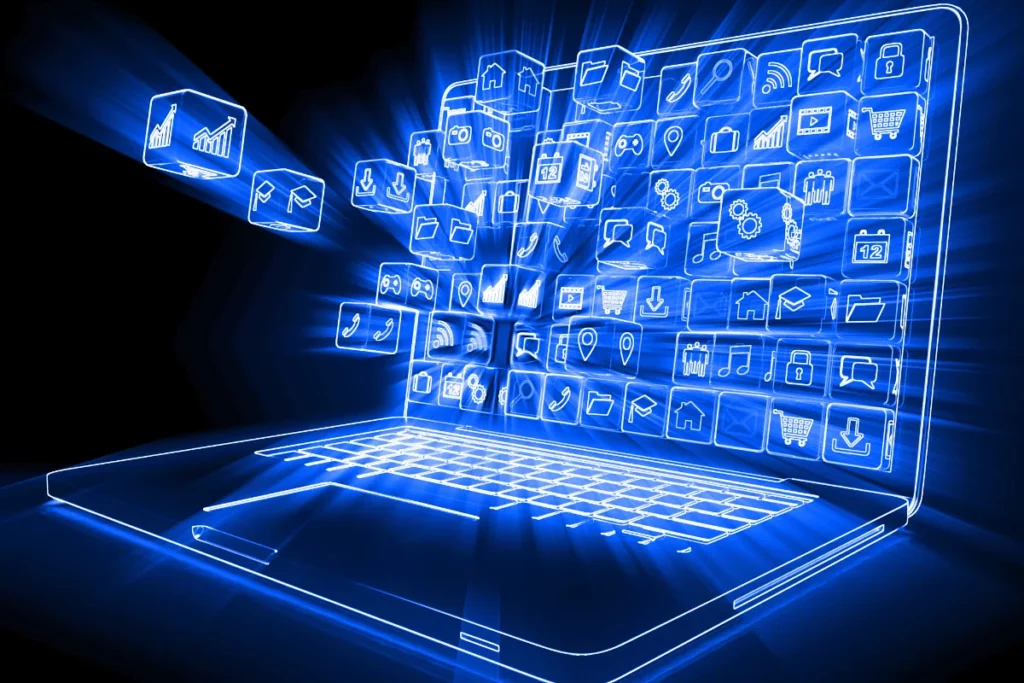
Frequently Asked Questions (FAQs)
Let’s wrap up this guide with some common questions on quantifying your qualitative data analysis:
What Industries Benefit Most From Qualitative Data Quantification?
Fields that thrive on user insights — tech, healthcare, finance — and any industry focused on customer experience can benefit. Quantifying feedback lets these sectors make user-centered improvements that matter.
What is the Role of AI in Qualitative Data Quantification?
AI is your fast research assistant who never gets tired or asks for coffee breaks. It tags, organizes, and even analyzes data in seconds, reducing tedious work and bias. AI brings up important insights that enable you to make faster, smarter decisions.
What Are Some Best Practices for Quantifying Qualitative Data?
Start with clear goals, keep your coding consistent, and pick tools that fit your needs (preferably AI-powered). Equally important, check your categories often to ensure they match what users are actually saying. And remember, a bit of patience goes a long way toward getting real, useful insights.

Conclusion — How to Turn Qualitative Data into Quantitative
Quantifying qualitative data turns prose into math. When you do it right, you bring a new level of clarity to your research. Plus, you present insights that everyone — from fellow researchers to the C-suite — can instantly understand and act on.
The best part? Quantification doesn’t have to lose the richness of your data. Instead, it tags and counts the insights that matter, making them surface and inform your product development.
Ready to turn feedback into fuel for better product decisions?
Create your free account with Marvin and let it transform your qualitative data into meaningful insights — beautifully organized, just a click away.