“How can we make user experience less intimidating for people looking to become researchers?”
“How do we help people understand AI-based research methods and tools?”
These are the questions Kate Moran works to answer as Vice President of Research and Content at Nielsen Norman Group (NN/g). As an expert on how AI applies to UX, she has a deep understanding of AI-based research products.
She spoke to our co-founder and CEO, Prayag Narula, about all the ways researchers can safely use these AI products and tools in their research.
Read on for key takeaways from the webinar, or listen to the entire conversation.

AI’s Impact on UX Research
Kate believes the first wave of AI-based tools was rushed, using AI more as a marketing buzzword than an effective feature. We are now seeing better and more thoughtfully crafted tools that help researchers work more efficiently.
For example, Kate uses AI to accelerate her research analysis. Or to check if she might be missing anything.
“We have good ReOps at NN/g. We have good templates and things that speed up that process. I still use Chat GPT to help me prepare for a study because there’s a lot of kind of tedious documentation. It is kind of helpful as a brainstorming partner.”
The flip side of better-integrated AI is unrealistic claims about what these tools can do. For example, some products claim that synthetic users can replace human users in research, while others claim AI can handle a bulk of the research itself.
“But why?” Kate asked.
Why are we so keen to do the user research without the users or the researchers?
Prayag pointed out that LLMs are tailor-made for synthesis and analysis. We should leverage these capabilities to make research more effective, useful, faster, and less expensive.
UX research can be complicated, messy and sort of esoteric, especially to business leaders. Kate worries that grand claims might encourage stakeholders to completely replace researchers or users with AI.
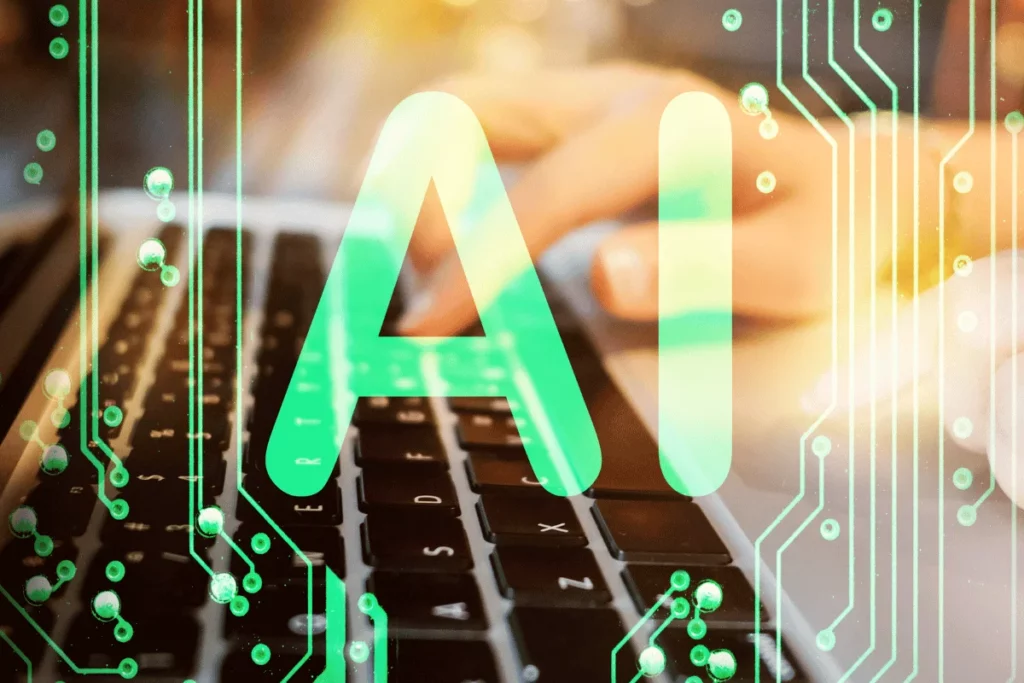
AI: Your New Research Intern
Instead of trying to bypass researchers and users, experts say we should think of AI as a research intern or assistant.
Kate explained, “Imagine this is an intern, and they know nothing about you. And so you have to tell them a lot about the product you’re working on, the dynamic of your team, and your constraints.”
Products such as Marvin use AI-powered features that are already trained on your research repository. They are more powerful as you need to provide less context.
But if AI can play the role of a research intern, will that make it harder for people looking to enter UX to land their first role?
“Our ability to think about context and nuance in really complicated ways, to me, is essential for being successful in UX research, design, product, or any other adjacent roles,” Kate said.
She has not yet seen AI tools be able to draw insights from scattered information. Researchers also weigh pros and cons for the business, users and dev teams before making a decision.
Kate hopes that companies will still see the value in hiring people as interns. Not only is it a longer-term investment, but AI also has its limitations. She wants to train people and help them do the advanced, creative, and contextual thinking that AI tools struggle with.
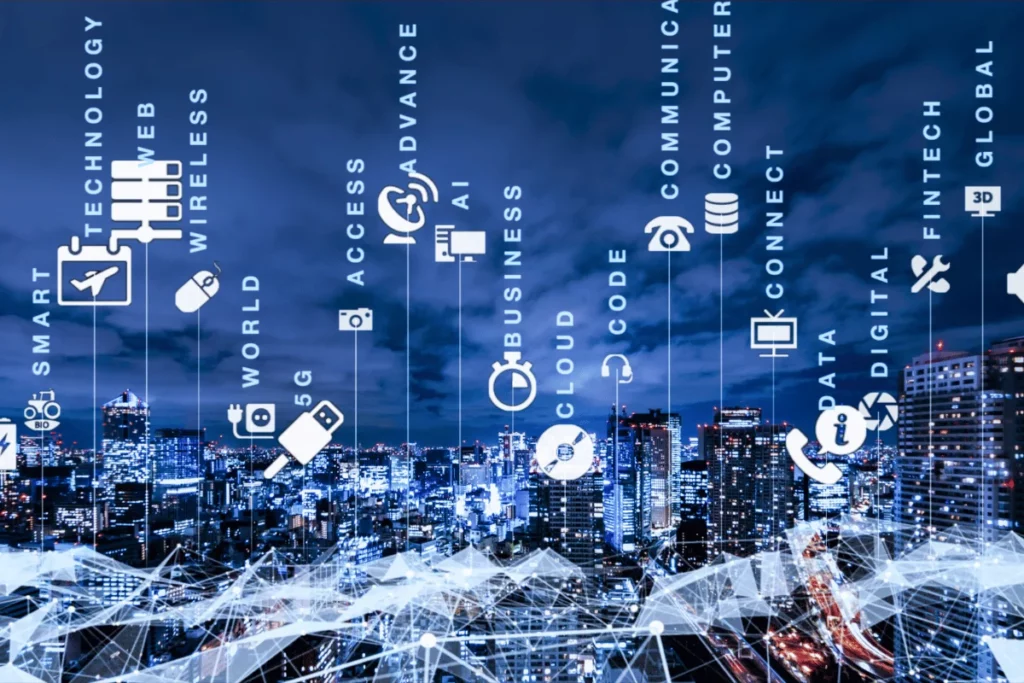
New AI-enabled Research Methods
New AI tools have also contributed to different ways of doing research. Several companies are working on survey-centric tools. These are some of the features they offer:
- Custom or real-time follow-up questions
- Option for participants to answer questions verbally
Kate believes we’re moving toward an asynchronous interview model that’s not quite a survey. These features are even more valuable to researchers as they can do it at scale. These tools will impact everything from sample sizes to research methodology.
While researchers use AI, so do the participants. Kate mentioned she’s noticed ChatGPT responses on surveys and diary studies. This is a problem for panel providers because there’s no effective way to check if a participant used AI to answer a question.
“The way that we’ve been identifying it is like, the answers are too complete, they’re too polished, they’re too excited.”
Read this guide for our top picks of survey analysis software.
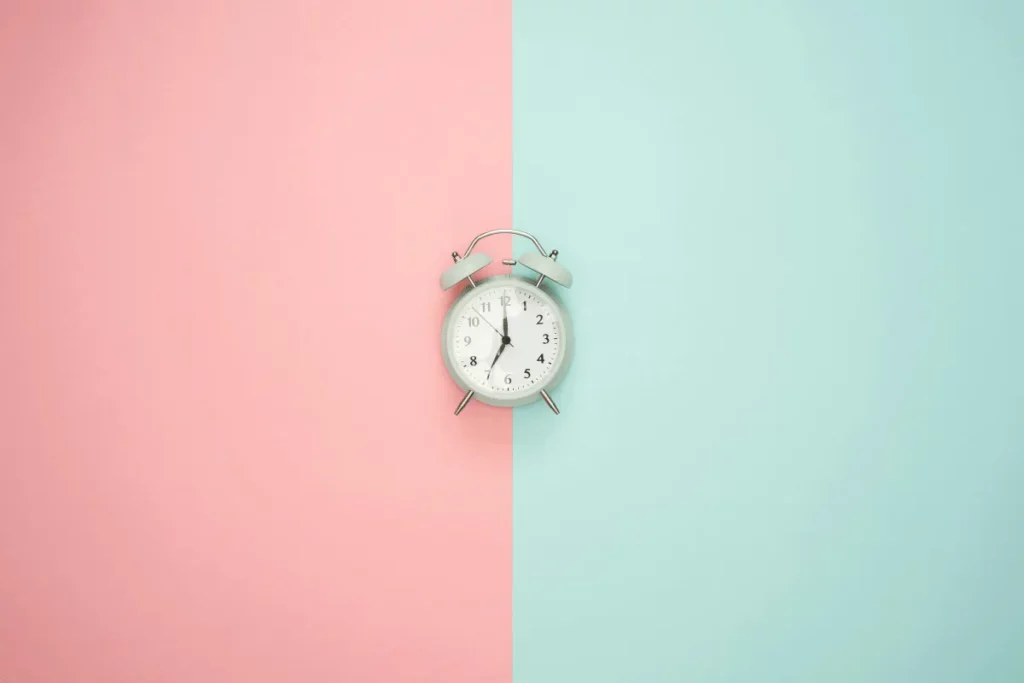
The Allure (And Challenges) of Synthetic Users
Companies often approach UX training and consulting firms such as NN/g for advice on synthetic users. Kate worked with Maria Rosala, Director of Research at NN/g, to compare different types of synthetic users to actual real research with human beings.
The Benefits
Despite her apprehension about such products, Kate found they could be useful in certain applications. Some of these tools are trained extensively on the following sources:
- Internet sources
- Books
- Published research
- Psychological research
These products can provide a great context for desk research. For example, Maria had a client project in a domain that was completely new to her. It was very complicated, and she didn’t have the requisite context to engage with these clients. She used synthetic users to test out her discussion guides. She was then better prepared for the actual research.
The Caveats
Kate warned, though, “It’s very dangerous and very likely that in organizations that don’t already value research, this is going to feel like good enough.”
She likened responses from synthetic users to junk food. It feels good but it is empty calories. Kate found that synthetic users tend to answer questions very differently from human beings. An AI model pretending to be a university student might say, “Yes, I always do all of my homework, and I do it on time. I never pull all nighters.” But in reality, that’s not how humans behave.
Companies do user research to understand users, their needs, workflows, and mental models better. But “If you can just ask a synthetic user a question, so can your competitors,” Kate said.
The Challenges
“I was always drawn towards the unexpected. The out-of-the-blue answer that I was not expecting,” Prayag said about when he did research. “Unlike quantitative research, qualitative research lives on the fringes. This one person who said something really interesting and then got validated. I don’t know if I’ve ever heard an LLM say anything super surprising to me.”
Kate explained why: “You could think about our footprint. Our records that we have of our society that are on social media, the Internet, and in published academic journals or novels. Is that the same as real life? Not usually.”
Shared this example:
“Have you ever written a review on Google for a gas station or a petrol station? If you have, I’m willing to bet it’s because it was a horrible experience, and you were mad. If I were to try to interview a synthetic user about their experience at gas stations, maybe it would just tell me horrible things because that’s all that is available on the Internet.” So, it’s not really a realistic portrayal of gas stations or human behavior.
Quite a few organizations are working to create synthetic users based on actual research companies did with human beings. That is more useful as it builds a persona researchers and stakeholders can use.
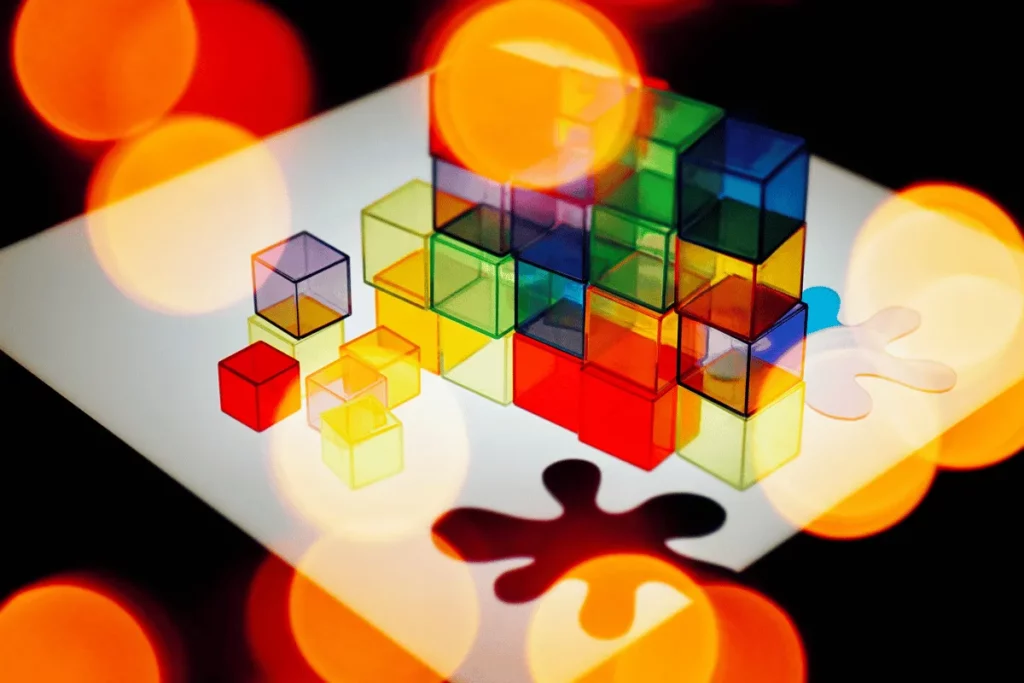
Personalization of User Experiences
“Design AI, I think, has been one of the most exciting things to think about in terms of how it changes UX because it’s changing the way we do our jobs.”
Kate said most experts speculate that design AI will reach the point where interfaces will be created in customized ways for individuals in real-time. Companies are seriously evaluating how to integrate this with products. Kate wrote about it in more detail in an article with Sarah Gibbons, Vice President at NN/g.
This is going to change what it means to design and who is doing the design work. We can think of this as putting personalization on steroids and doing it to an extent we’ve never been able to do before.
AI enables the mining of large sets of customer data to understand it at an unprecedented scale. It can also be used to make some decisions on how to display things.
For example, a challenge with content design is that everybody has a different background knowledge. Kate’s articles might be read by an expert with 30 years of experience or someone reading their first ever UX article. With design AI features, she could write a piece that not just serves different readers, but one that’s interactive too.
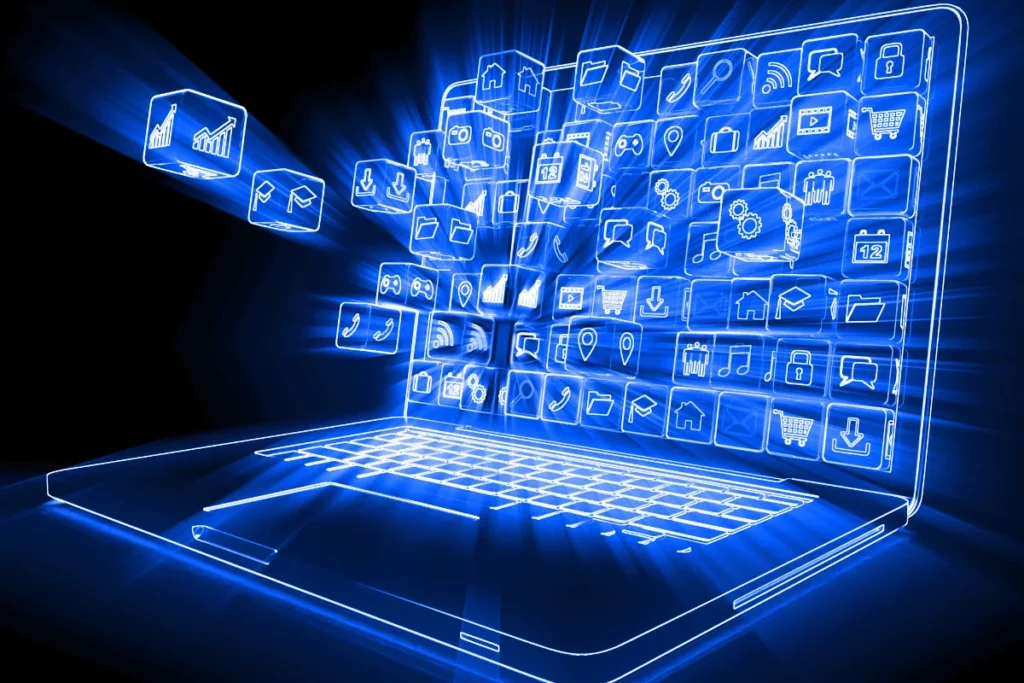
Always Double-check AI
No matter how you use AI, everyone has a responsibility to check the output. People tend to believe AI-generated answers because they sound realistic. But that’s what LLMs are good at.
“If you’re asking AI what research methodology you should use next and you don’t have a research background, it’s probably a good idea just to double-check before you get started,” Kate advised.
It’s also a good way to think about how to work with AI because you need to provide specific prompts. Instead of asking AI to create a research plan, break it down into steps. Start with providing context about the goals and asking which methodology and how many participants work best. To help write screening questions, create a template. (This guide on how to craft UX research questions will give you some good tips!)
The key to working with LLMs is to give them guardrails and constraints. If you combine it with checking the output, AI can help you avoid mistakes, bridge gaps, and work efficiently. Tools like Marvin are different, though. AI within a product is much more contextual and requires less handholding.
“But we still do need to double-check,” Kate added.
Hot Tip: Marvin provides references for all the AI analysis it generates so you can easily check the information.

AI Adoption at a Personal Level
Kate was an English major who has worked as a technical editor and front-end developer. She also has a master’s degree in information science. She found that communication was a key skill in all of her degrees and roles.
Even though she works with AI products on a daily basis, she doesn’t use it to help her write. While she considers herself a good prompter who follows AI advice, she can’t get a result she likes better than her own style. But that’s because it’s one of her skill sets.
On the other hand, she’s more open to using AI for skills she’s just starting to learn. AI can fill skill gaps in areas you know you’re lacking, such as design or analysis.
People like to adopt AI-native products such as Marvin because they can help turn around projects quicker. You can use in-built modules to cut down repetitive work. Researchers can spend more time using their core skills to test extensively.
We loved what Kate said about doing good research:
“Curiosity should never be satisfied.”
At Marvin, we’re happy to play our part in keeping the curiosity alive. Want to see how? Sign up for a custom demo today.